Why is data science important for business?
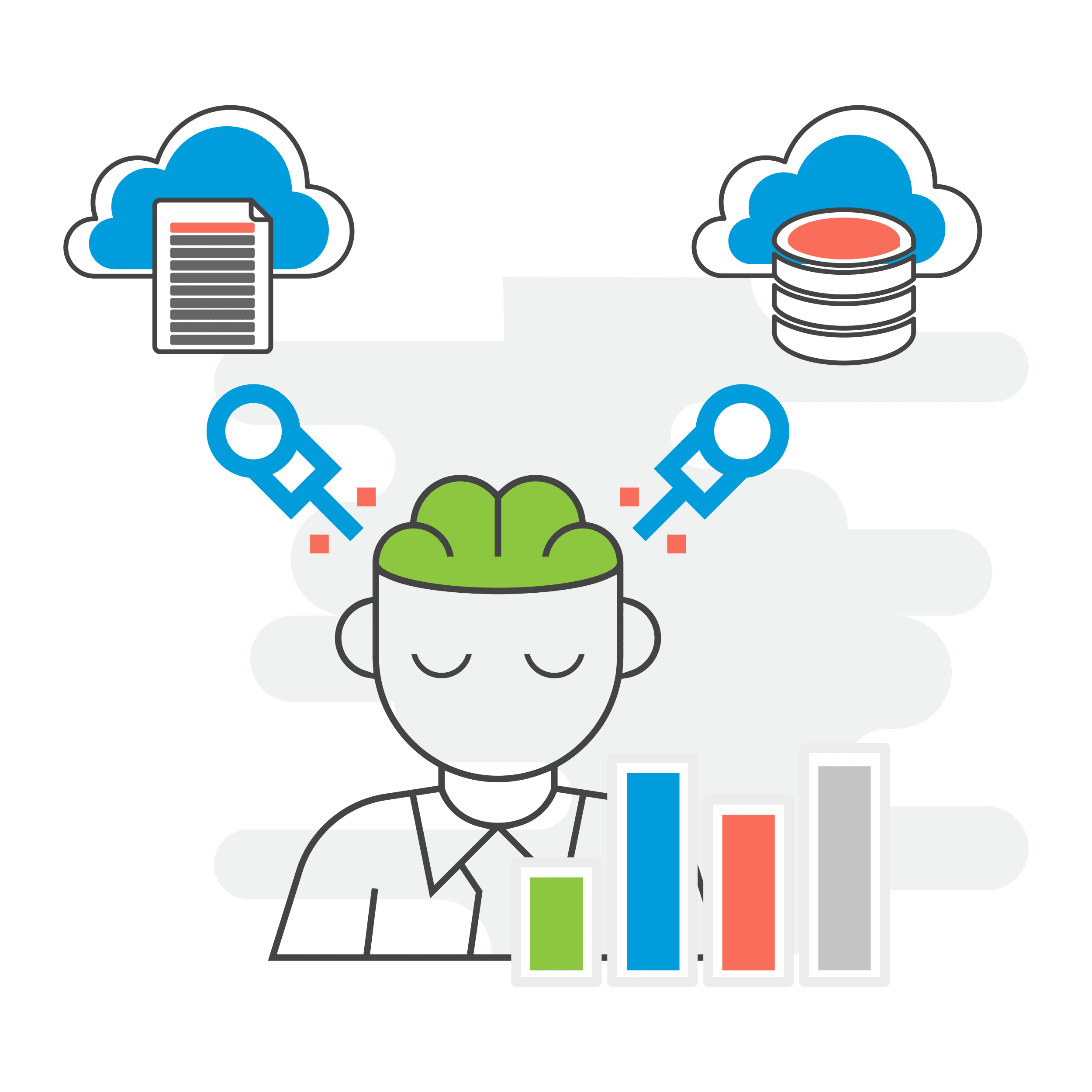
Your 101 guide for business
As enterprises increasingly turn to digital transformation, data science is becoming front and center to the discussion surrounding data analytics.
“Data science is the cutting edge of data analytics,” write Dr. Jay Boisseau and Dr. Lucas Wilson at CIO. “It’s a process of testing, evaluating and experimenting to create new data analytics techniques and new ways to apply them.” Not only that, but it is becoming central to enterprise operations. “Strong enterprise data cultures should include data scientists who continually strive to increase capabilities while working to enable the larger enterprise staff to use mature, proven analytics tools,” the researchers conclude.
With that mindset at the forefront, RTS Labs is dedicated to integrating the scientific approach that data science encourages to all the projects we undertake. And we want to enable you to do the same. In this brief guide to data science, you’ll learn:
- Why data science is important to modern enterprises,
- How it’s being used across industries to transform business, and
- The steps and core competencies involved to integrating data science into your enterprise.
You can learn more about what RTS Labs is doing with data science for enterprises on our data science consulting page. Otherwise, let’s jump in.
Data science: What it is and why it’s important
The form and function of data science run together: data science turns raw data into accurate predictions so you can make high-impact decisions that lead to competitive advantage. And with our lives increasingly driven by data, using data science has become a focal point for organizations of all types.
Traditional business intelligence is nothing new. But it only tells you what a problem is, along with a few details. In contrast, data science reveals the source of a problem and can make relatively accurate predictions for where and when the same problem could crop up in the future. It’s like holding the winning lottery ticket.
Behind data science is raw data — the material that feeds everything. Big data is typically defined by “the seven V’s”: volume, variety, velocity, value, variability, veracity, and visualization. These answer how much data is available, how fast it can be accessed, where it comes from, how it can be used and more.
Most of the “v’s” have to do with with the form of data. But two parts (visualization and value) have more to do with the function of data for the enterprise. Visualization graphs can be integrated to analyze various data sets, giving you fresh and valuable insights, even in real-time. For example, you could know the implications of real-time customer purchases. This is where enterprises get the value of big data from.
Why data science is important
Ninety percent of the world’s data was created in only the last two years — we’re in the middle of the digital revolution. The amount of data being generated daily is staggering: 2.5 quintillion bytes! If the bytes were converted to pennies, it would be enough to cover the earth twice a day.
The US alone creates 2,657,700 gigabytes of Internet data every minute. For every minute you spend reading this article, YouTubers will have watched 4.14 million videos. WhatsApp hit 100 million calls per day a long time ago.
You get the picture. We’re dealing with a lot of data these days. And enterprises can harness that data for growth.
While data can reveal business insights, most companies are completely unprepared for the challenge. With the right preparation, data tools can solve complex business problems:
- Predicting future demand with predictive analytics
- Recommending buyer options with recommendation engines
- Optimizing marketing campaigns with tactical optimization
- Detecting fraud with automated decision engines
- Understanding customers better with nuanced learning and natural language processing
Data science and DevOps for the modern enterprise
Data science and DevOps can work beautifully together. Both place an emphasis on closing the feedback loop between teams to achieve continuous improvement across systems, processes and outcomes. And both can make for lightweight operations, particularly when run on Docker within containers.
Obviously DevOps and data science each have their own focus as well: data science projects focus on filtering, extracting and formatting data for more effective use, while DevOps projects focus on bridging technology operations and development for better quality assurance and outcomes. Even in their differences, one can see the similarity: when done well, both DevOps and data science projects both depend on and improve the entire enterprise.
Because of this dependency and impact, projects work best when DevOps and data science teams work together. “DevOps involves infrastructure provisioning, configuration management, continuous integration and deployment, testing and monitoring,” writes Janakiram MSV at Forbes.
As organizations prepare to take full advantage of the data available to them (whether in the form of data analytics or machine learning), operators will increasingly work with both developers and data scientists (and data engineers). “Data engineering, a niche domain that deals with complex pipelines that transform the data, demands close collaboration of data science teams with DevOps,” Janakiram concludes.
How data science and DevOps work together
Data scientists use huge amounts of information to find and predict problems, along with insights into and correlations between nodes. The intel they gather can drastically improve DevOps’ efforts. In turn, DevOps can help data science teams develop a containerized approach to their data projects.
Right now, integrating data science and DevOps at the enterprise level will position organizations ahead of the curve. Soon, informing DevOps projects with data and data projects with a DevOps approach could very well become the norm. Why not position yourself with the experts?
Where RTS Labs brings data science expertise
RTS Labs works within a host of industries to bring DevOps and data science expertise into enterprise operations. Here are some of the data-driven challenges industries face and how data science can help solve them.
Healthcare
While it’s not true across the board, many organizations in the healthcare industry struggle with efficiency. Data science services can help physicians make better treatment decisions and recommend more effective preventative care — not to mention more accurate and faster billing.
Uses for data science in healthcare include:
- Claims review prioritization
- Medicare/Medicaid fraud prevention
- Medical resources allocation
- Alerting and diagnostics from real-time patient data
- Prescription compliance
- Physician attrition improvement
- Survival analysis
- Medication and dosage effectiveness
- Readmission risk
Finance
There are incredible amounts of data available to the financial sector. This enables companies to offer credit online with less risk, such as loans for start-up entrepreneurs. Using data, companies can create a baseline for spending patterns, and identify when something abnormal happens to prevent fraud.
These are just some of the applications of data science consulting within the financial industry:
- Credit card fraud prevention
- Credit risk analysis
- Treasury or currency risk analysis
- Organizational fraud detection
- Accounts Payable recovery
- Anti-money laundering efforts
- Efficient debt collection
E-commerce & retail
Data science helps both retail stores and eCommerce brands better understand their customers. This makes it easier to gauge creditworthiness, facilitate accurate pricing, upsell and make personalized suggestions.
Consumer brands are using data science services to improve:
- Pricing and increasing Average Order Volume
- Merchandising
- Personalization
- Inventory management
- Warranty analytics
- Location of new stores
- Product layout in stores
- Shrinkage analytics
Non-profit
Nonprofit organizations need resources to achieve their mission. But to attract donors, they must prove their worth by showing the result of their work. Data science can help nonprofits acquire funds and do more good — all with better informed decisions.
Nonprofits can use data science services to:
- Optimize fundraising
- Identify and target groups
- Discover relationships
- Develop incentives
- Measure performance of activities
- Optimize relief efforts
- Tackle issues related to education, healthcare, public safety and the environment
- Allocate funds appropriately
Logistics
Logistics management requires an accurate view of constantly changing variables: shifting demand, human error, traffic, fuel costs, and changes in the weather, to name a few. Logistics managers can apply predictive to all of these challenges, enabling companies to experience less mechanical downtime and more efficient routes.
Logistics companies can use data science consulting in:
- Managing demand forecasting
- Order picking from existing stocks
- Replenishment procurements to keep stock levels adequate
- Packaging for efficient delivery
- Routing of packages to avoid choke points
HR management
Finding the right people is the key to growing your business. While it’s an ongoing challenge to recruit, hire, train, and manage them, data science can help. Data science consulting and data analytics can improve:
- Resume screening
- Employee churn
- Training recommendation
- Talent management
- Tracking work hours
- Detecting employee theft
- Call center/call routing
- Call center message optimization
- Volume forecasting
- Staff rostering
6 steps for utilizing data science
Taking on a data science project is not a laissez-faire undertaking. You should identify the problems you are trying to solve, define data gathering methods and analytical tools and more. These are the six steps we recommend for any data science project — along with critical questions to ask, steps to take and tools to use.
Step 1: Define the problem
The first step to solving a problem is defining it. Then you must translate your questions about the data into something actionable. For example, in retail you should ask:
- Who are the customers?
- Why do they buy?
- How do we predict if they’ll buy?
- What’s our return-on-investment for increasing sales?
Step 2: Collect raw data
Here you must determine what data you have, what you need and how you’re going to get it. You can use:
- Surveys
- Experiments for gathering qualitative or quantitative data
- Pre existing data in tables and databases
Step 3: Clean the data
Your data may be structured, but it can still be messy. And the quality of your output depends the quality of your input. The steps include:
- Eliminate common errors
- Watch for invalid entries
- Check for date range errors
- Data registered from before sales started
Step 4: Examine the data
This step where you search for the best ideas to test. And then decide what you think will turn into insights. This stage has a few steps of its own:
- Prioritize your questions
- Look for interesting patterns
- Consider irregularities
- Find commonalities
- Trace patterns for deeper analysis
Step 5: Perform in-depth analysis
Now you’re ready crunch the data and reveal some insights. For example, in retail you can create a predictive model that compares one group of customer data with a benchmark. Alternatively, learn which marketing channels are more likely to appeal to certain groups based on behavioral data that you’ve gathered.
This is where you need the right tools in place.
Step 6: Visualize the Data
Start communicating your data to staff, customers and stakeholders. Visualizing the data can help you:
- Find a compelling story idea
- Craft a story structure
- Tie your data in with the story
- Reveal the insights of the data
- Plan a narrative that shows how the problem is solved
- Motivate people to action
Getting started with data science for your business processes
Data science can improve nearly all of your business processes, from existing Salesforce projects to new enterprise applications built with DevOps. RTS Labs will help you realize all of its potential.
There’s no question data science is transforming modern business. The only question is how will you harness its power to make better high-impact decisions, build a better business with a competitive advantage?
Core competencies in data science
Any technology partner that you work with — as well as their data engineers and data scientists — should have core competencies in data normalization, data matching, attribution, and prediction.
Which area you focus on depends on both the enterprise problem you are trying to solve and the industry that you work in. Data normalization, for example, may be most important to an enterprise undergoing legacy modernization efforts, while a predictive modeling tool may be best for a new, digital native eCommerce brand.
Data Normalization
- Competency at all levels of data infrastructure – from foundational database design to cutting edge predictive analytics.
- Development and execution on crucial data governance and design decisions.
- The ability to ensure data integrity and to implement best-practice quality assurance.
- Data infrastructure with a focus on yielding high-level and in-depth insights into operational performance for both transactional and analytical systems.
Data Attribution
- Cataloging of cross-sectional details about your customers, products, and metrics.
- Analysis of conversion KPIs and outcome prediction utilizing advanced machine learning techniques.
- Analysis and visual interpretation of “customer conversion stories” or other qualitative data to assist key decision-makers and strategic development.
Data Segmentation
- Discovery and measurement of new cohort populations.
- Delivery of key insights from operational metrics and KPIs.
- Crafting of targeted and personalized marketing messages in order to reach untapped marketing segments.
- Identification of customers sub-groups, demographic cohorts, and consumer behavioral traits.
- Strategic analysis and development of core business insights – churn and customer retention analysis and customer profiles.
Time Series Analysis
- Analysis of trends and seasonality – analyze existing patterns to uncover ongoing trends and to predict future trends.
- Intervention analysis – determine whether manipulation of certain attributes will yield desirable outcomes.
Predictive Modeling
- Financial risk and fraud analysis/forecasting.
- Data-informed digital marketing and advertisements with tools for lead scoring, content targeting, and campaign optimization.
- Website traffic prediction – planning for peak hours and resource allotments.
- Geographic and demographic sales forecasting – consumer segments targeting.
Natural Language Processing
- General, content-based textual classification.
- Sentiment Analysis: Getting value from unstructured, textual data in customer forums or from social media.
- Topical keyword tagging: Automatic generation and application of content-based tags for articles and blogs.