Unleash the Power of Data & AI for Your Enterprise
Let us help you leverage data and AI as your strategic multiplier.
Harness the Full Potential of Your Data
To help you maximize your data’s full potential and make more informed decisions, RTS Labs provides a full spectrum of data and AI services. Because of our expert knowledge and extensive experience in the industry, we provide you with cutting-edge solutions that are uniquely suited to your needs, situation, and budget.
Your Vision, Backed by Our Experience
Data Strategy & Governance
Drive business success with a robust data strategy. Develop a holistic and well-articulated data strategy perfectly aligned with your business goals. We understand that data is a strategic asset that can drive business success. Our team works closely with you to establish a broad-based data strategy that addresses all your business needs. We also implement data governance frameworks to ensure data quality, integrity, and compliance. With our expertise, you can make confident, data-driven decisions that propel your organization forward.
Data Engineering & Integration
Unlock the power of your data with end-to-end solutions. Complete data engineering services can help you mine your data for insights and value. Our experienced team specializes in extracting, transforming, and integrating data from diverse sources. We create a unified view of your data, providing you with a comprehensive and accurate understanding of your business. By harnessing the power of data engineering, you can derive meaningful insights, optimize operations and processes, and make informed decisions that drive business growth.
Advanced Analytics & AI
Discover valuable insights with advanced analytics & AI. Leverage advanced analytics and AI technologies to uncover actionable insights and drive predictive and prescriptive analytics for detailed and timely information on customers, optimal product mix, and current and anticipated market conditions, and achieve cost reduction. Our team of experts is skilled in developing AI-powered solutions that automate processes, detect patterns, and make accurate predictions. By harnessing the power of advanced analytics and AI, you gain a competitive edge, identify new opportunities, and optimize your business operations for maximum efficiency and profitability.
Data Visualization & Reporting
Cloud-based Data Solutions
Unleash the power of the cloud for scalable data solutions.
Utilize the scalability and security of cloud platforms like Azure and AWS to build dependable data solutions that are customized to serve your specific business needs. We help you migrate to the cloud, design data lakes and warehouses, and optimize your data infrastructure using serverless computing and managed services. With our cloud-based data solutions, you can efficiently store, process, and analyze large volumes of data, enabling you to scale your business and discover new opportunities.
Leveraging Your Data & AI Advantage
-
Data-Driven Decision-Making
Gain actionable insights for informed decision-making, identifying trends, and predicting outcomes to drive strategic choices.
-
Enhanced Experiences
Understand customers better, personalize experiences and deliver targeted offerings for improved satisfaction and loyalty.
-
Operational Efficiency
Streamline processes, automate tasks, and optimize resource allocation, enhancing productivity and maximizing outcomes.
-
Competitive Advantage
Stay ahead of the competition by leveraging data-driven insights, capitalizing on opportunities, and outperforming rivals.
-
Risk Mitigation & Compliance
Proactively address risks, detect anomalies, and ensure compliance with industry regulations, safeguarding your business and maintaining trust.
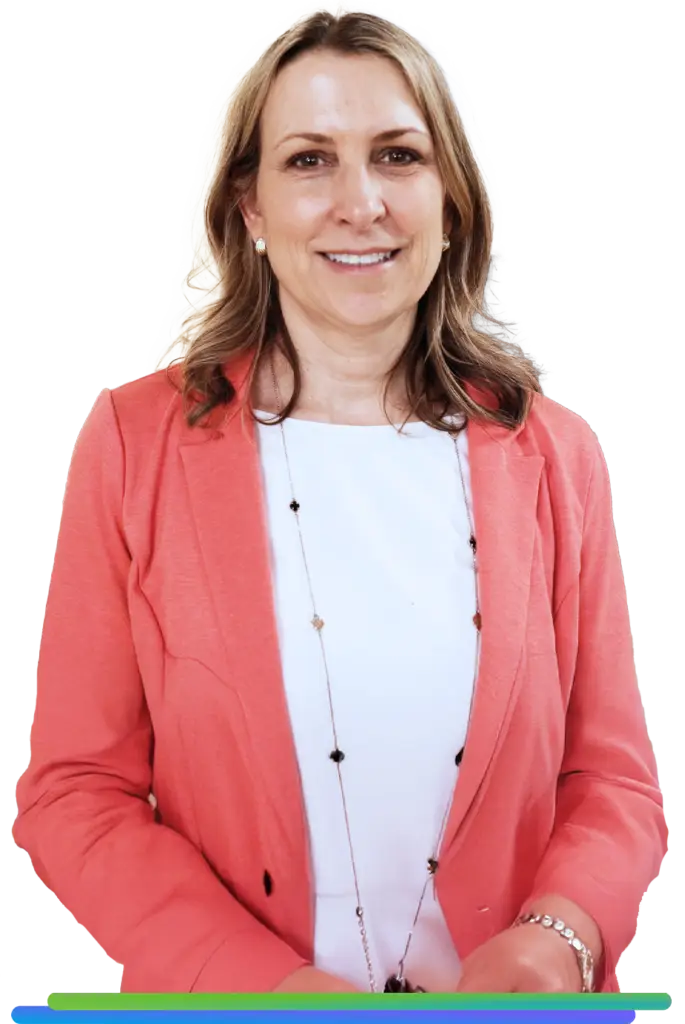
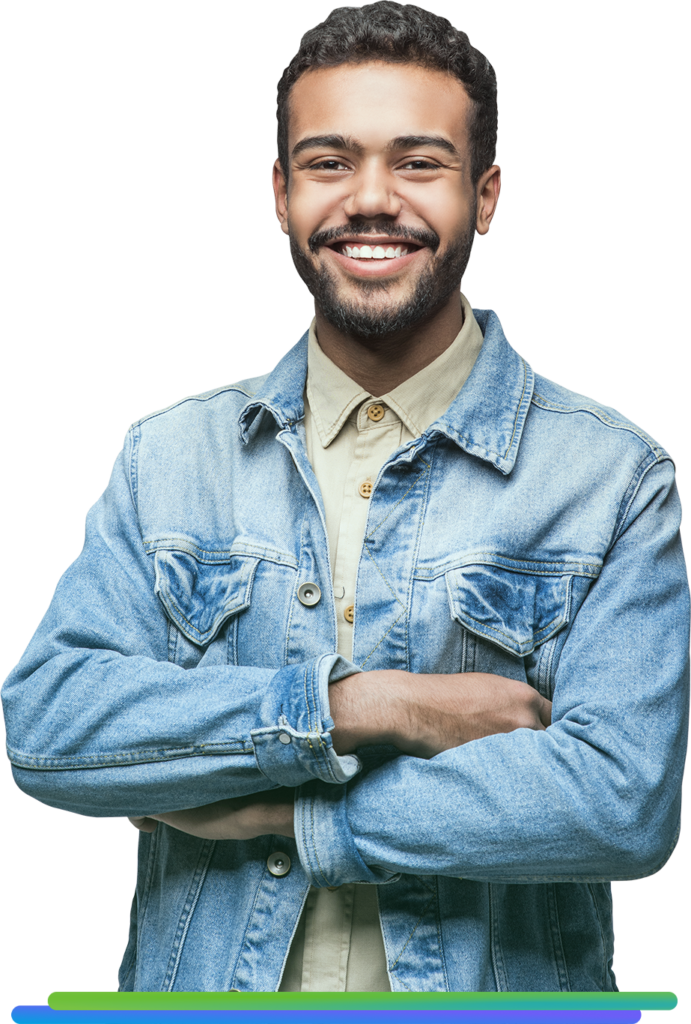
Why Choose RTS Labs?
-
Deep Expertise in Data & AI
Our team of data scientists, AI experts, and technology professionals possess extensive knowledge and stay updated with the latest advancements, delivering cutting-edge solutions tailored to your unique business needs.
-
Customized Solutions
We understand that every business is unique. Our solutions are customized to address your specific challenges and drive maximum value for your organization.
-
Proven Track Record
We have a successful track record of helping businesses unlock the power of data and AI, delivering tangible results, and driving fast business growth for our clients.
-
Collaboration & Partnership
We work closely with you, fostering collaboration and partnership throughout the process. Your success is our priority, and we strive to exceed your expectations.
Customer Success Story:
eCom Engine
eComEngine’s tools help Amazon sellers with feedback management, inventory forecasting, and reputation monitoring, all of which enhance selling performance on the Amazon platform.
let’s talk
Unleash the Power of Data and AI for Your Business!
Contact RTS Labs today to start your transformative journey toward data-driven innovation and success. Our data experts are ready to help.