Enhancing the Value of GenAI with Domain-Specific Data
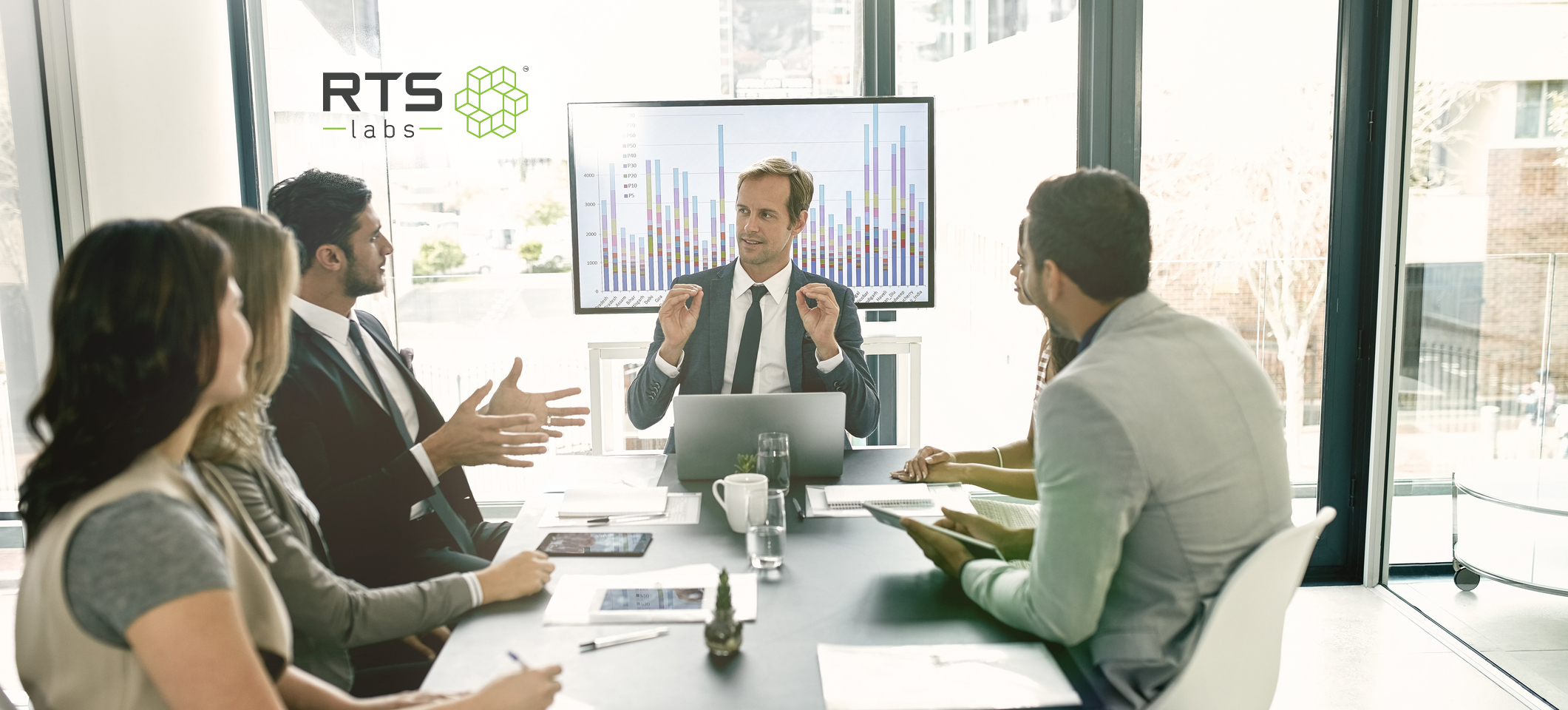
In an era where artificial intelligence is transforming industries, businesses are looking for ways to maximize the value of their AI investments. Generative AI (GenAI) holds immense potential for businesses, but it’s true value is realized when it is tailored to the specific needs of an industry. By integrating domain-specific data into GenAI models, companies can elevate their AI performance, improve relevance, and drive better business outcomes.
In this article, we’ll explore how using domain-specific data can enhance GenAI’s capabilities and provide actionable steps to help businesses get started.
Understanding Generative AI and Its Limitations with Generic Data
Overview of Generative AI
Generative AI refers to artificial intelligence models that can create new content based on patterns they learn from the data they’ve been trained on. Popular examples of GenAI include models like GPT-4, known for its ability to generate human-like text, and DALL·E, which creates original images from text descriptions. These models can automate various tasks, including content generation, language translation, and data analysis, making them a valuable tool for many businesses.
However, like all AI models, GenAI is only as good as the data it’s trained on. When trained on generic datasets, GenAI can provide impressive results, but these results may lack the depth and accuracy required for specialized industries.
The Challenges of Using Generic Data
While GenAI models trained on generic data can be useful for broad applications, they often fall short when applied to specific industry needs. For example, a model trained on general internet data may produce irrelevant or inaccurate results when tasked with generating technical documentation for a specialized field like medicine or law.
Some common challenges include:
- Irrelevant outputs: AI models trained on generic datasets may fail to understand the nuances of a specific industry.
- Inaccuracy: Lack of domain-specific knowledge can lead to errors, which is particularly concerning in fields like finance, healthcare, or legal services.
- Risk of hallucination: GenAI models may produce convincing but inaccurate information, which can be detrimental in high-stakes industries.
Importance of Domain-Specific Data
Domain-specific data is critical for businesses that need precise and contextually relevant outputs. By training GenAI models on data specific to a particular industry, businesses can improve the accuracy, relevance, and reliability of their AI-generated content.
The Benefits of Integrating Domain-Specific Data with GenAI
Improved Accuracy and Relevance
When GenAI models are trained using domain-specific data, their outputs become much more accurate and relevant to the business’s needs. Instead of relying on generalized knowledge, these models draw from information directly related to the industry, allowing them to make informed decisions and generate outputs that align with real-world applications.
For instance, a GenAI model trained with medical-specific data will be better equipped to generate accurate diagnoses or treatment recommendations, whereas a financial GenAI model will provide more precise risk assessments or investment strategies.
Enhanced Personalization
Personalization is critical to customer engagement, and GenAI models integrated with domain-specific data can offer highly tailored experiences. In industries like e-commerce or marketing, AI models can analyze customer behavior and preferences, then use this data to generate personalized product recommendations, content, or marketing messages that resonate on a deeper level.
Businesses can leverage domain-specific data to:
- Enhance user engagement by creating content or experiences that reflect the customer’s needs and preferences.
- Drive higher conversion rates by offering more relevant solutions to potential customers.
Better Decision-Making and Insights
Domain-specific data can also help businesses make better, more informed decisions. GenAI models trained with specialized data can provide actionable insights that are grounded in industry knowledge, leading to more accurate predictions and better business outcomes.
For example:
- Healthcare: AI models trained with medical records and clinical data can offer insights into patient outcomes and help healthcare providers make better treatment decisions.
- Manufacturing: GenAI models trained on supply chain and production data can optimize processes, predict equipment failures, and reduce operational costs.
Competitive Advantage
Companies that harness the power of GenAI with domain-specific data gain a significant competitive advantage. By offering more accurate, reliable, and industry-relevant solutions, these businesses are better positioned to meet customer needs, streamline operations, and stay ahead of competitors.
For instance, businesses in the legal field can use AI models trained on legal precedents and case law to offer more precise legal advice, while those in the finance industry can enhance portfolio management and risk assessment with financial-specific data.
Steps to Enhance GenAI with Domain-Specific Data
Identifying Relevant Domain-Specific Data
The first step in enhancing GenAI with domain-specific data is identifying the right data sources. Not all data is equally valuable, and businesses must determine what types of information are most relevant to their industry and use cases.
Key considerations include:
- Proprietary databases: Internal data such as customer records, transactional data, and operational data.
- Industry-specific datasets: Publicly available datasets that provide information relevant to your field, such as government reports or research studies.
- Third-party data sources: Data that can be purchased or licensed from data providers specializing in your industry.
Data Collection and Preparation
Once the relevant data sources have been identified, businesses must collect and prepare this data for use. This step involves ensuring that the data is clean, properly formatted, and free from errors or biases that could negatively impact AI model performance.
Best practices for data collection and preparation include:
- Data cleaning: Removing duplicates, errors, and irrelevant data points.
- Pre-processing: Standardizing data formats and ensuring consistency across datasets.
- Validation: Ensuring the data is accurate and up-to-date before using it for AI model training.
Training and Fine-Tuning AI Models
After the data is prepared, it’s time to integrate it into the GenAI model training process. This often involves using techniques like transfer learning, where a pre-trained GenAI model is fine-tuned with domain-specific data to adapt it to the unique requirements of an industry.
- Transfer learning: Allows businesses to leverage existing AI models and adjust them for their specific domain without starting from scratch.
- Fine-tuning: Involves tweaking the model’s parameters to optimize its performance with the specialized data.
Testing and Validation
Testing the AI model’s performance is a crucial step to ensure it produces reliable, industry-specific results. Businesses should evaluate the model’s accuracy, relevance, and usability across various scenarios to confirm its effectiveness.
Regular validation and continuous improvement are necessary to ensure that the model adapts to changing data and evolving industry trends.
Case Studies: Success Stories with GenAI and Domain-Specific Data
Company A: Improving Healthcare with Specialized AI Models
A healthcare company used domain-specific data to train its GenAI models, focusing on medical research, patient records, and clinical trial data. As a result, the company improved diagnostic accuracy and offered personalized treatment recommendations based on each patient’s unique medical history.
Company B: Financial Services Firm Optimizes Risk Management
A financial services firm integrated proprietary data into its GenAI models to enhance risk assessments. By using financial-specific data, the AI could more accurately predict market trends, assess potential risks, and recommend strategies for mitigating financial exposure. This resulted in more reliable decision-making and better regulatory compliance.
Key Lessons Learned
Both companies faced challenges such as data integration issues and ensuring data quality, but by addressing these challenges head-on, they successfully implemented GenAI models that provided significant business value. The common theme across these cases was the importance of domain-specific data in achieving more accurate, reliable, and actionable AI outputs.
Overcoming Challenges in Implementing Domain-Specific Data
Data Availability and Accessibility
One of the biggest challenges businesses face is accessing high-quality domain-specific data. Many industries have limited data availability, and obtaining the right data can be time-consuming or expensive. Businesses can overcome this by forming partnerships with data providers, using publicly available datasets, or generating synthetic data when real data is unavailable.
Data Privacy and Compliance Concerns
When using domain-specific data, businesses must also navigate the complex landscape of data privacy regulations. Industry-specific laws like GDPR, HIPAA, and CCPA require companies to handle sensitive data carefully. Ensuring AI models remain compliant with these regulations is critical to avoiding legal penalties and maintaining customer trust.
Ensuring Data Quality and Consistency
For GenAI models to perform well, the data used must be of the highest quality. Data that is inconsistent, outdated, or biased can lead to inaccurate outputs, undermining the AI’s effectiveness. Businesses should implement robust data validation and auditing processes to ensure data quality.
Scaling and Maintaining AI Models
As industries evolve, businesses must continuously update their GenAI models with new domain-specific data. This process requires scaling the models to handle larger datasets and adapting them to changing industry standards and trends. Maintaining AI models involves ongoing monitoring and retraining to ensure they continue delivering valuable insights.
The Future of GenAI with Domain-Specific Data
Emerging Trends in Domain-Specific AI
The future of GenAI lies in specialization. AI models will increasingly be tailored to specific industries, with advancements in areas like legal tech, fintech, and healthcare AI. As businesses continue to demand more personalized and industry-relevant solutions, the use of domain-specific data will become a standard practice.
AI Regulations and Data Governance
With the rapid growth of AI, future regulations are likely to address how domain-specific data is used in AI model training. Businesses must stay ahead by adopting proactive data governance practices and ensuring their AI models comply with ethical standards.
Preparing Your Business for the Next Evolution of AI
Businesses that invest in domain-specific data now will be better positioned to adapt to the next evolution of AI. Leadership will play a crucial role in fostering a culture of innovation, compliance, and ethical AI development, ensuring that companies can thrive in a rapidly changing technological landscape.
Frequently Asked Questions (FAQ)
1. What is Generative AI (GenAI) and how does it differ from other types of AI?
Generative AI refers to a subset of artificial intelligence models designed to create new content, such as text, images, music, or code, based on patterns learned from large datasets. Unlike traditional AI, which focuses on identifying patterns or making predictions, GenAI generates new outputs, like how GPT-4 can write articles or DALL·E can create images from text prompts. The main distinction is that GenAI can produce original content rather than simply analyzing existing data.
2. Why is domain-specific data important for GenAI?
Domain-specific data enhances the relevance, accuracy, and utility of Generative AI models by training them on information that directly relates to a particular industry. This allows AI to generate more contextually relevant and accurate outputs, which is especially important for businesses in fields like healthcare, finance, or legal services, where precision and specialization are crucial.
3. How can my business acquire domain-specific data?
Businesses can acquire domain-specific data through various channels, such as:
- Proprietary data: Using internal data collected from business operations, customer interactions, and historical records.
- Industry-specific datasets: Leveraging publicly available datasets or research reports relevant to your industry.
- Third-party providers: Purchasing or licensing data from specialized data vendors who offer curated, domain-specific datasets.
4. What are the challenges of integrating domain-specific data into GenAI models?
Some common challenges include:
- Data availability: High-quality, domain-specific data can be scarce or expensive.
- Data privacy and compliance: Using sensitive or proprietary data requires adherence to regulations such as GDPR, HIPAA, or CCPA.
- Data quality: Ensuring that the data is accurate, consistent, and free from biases is critical for producing reliable AI outputs.
- Scalability: Continuously updating and scaling models to handle evolving datasets and industry needs can be complex.
5. How do I ensure my GenAI models are compliant with data privacy regulations?
To ensure compliance with regulations like GDPR, HIPAA, or CCPA, businesses should:
- Use secure data storage methods to protect sensitive information.
- Implement anonymization techniques where possible to prevent the exposure of personally identifiable information (PII).
- Regularly audit data usage practices to ensure that AI models align with legal standards.
- Seek legal counsel or involve compliance officers during the AI development process.
6. What is the role of fine-tuning in enhancing GenAI with domain-specific data?
Fine-tuning involves taking a pre-trained GenAI model and adjusting it with domain-specific data to better fit the unique requirements of your industry. This process allows businesses to leverage the general knowledge already embedded in the model while adapting it to generate more accurate and relevant outputs for specialized tasks.
7. How often should GenAI models be updated with new domain-specific data?
AI models should be updated regularly as new domain-specific data becomes available or when industry standards evolve. Continuous updates help the models stay relevant and accurate. The frequency of updates depends on factors such as changes in market conditions, regulations, and the availability of new data sources. Implementing a system for ongoing monitoring and feedback collection can help determine when updates are necessary.
8. Can domain-specific GenAI models be scaled for larger datasets or new business needs?
Yes, GenAI models can be scaled as new data becomes available or as the business’s needs evolve. Businesses can achieve this by:
- Expanding the dataset size to include more comprehensive domain-specific information.
- Continuously fine-tuning the model with updated data to keep it relevant.
- Utilizing cloud-based infrastructure to handle larger datasets and more complex AI workloads, ensuring the model remains efficient as it grows.
9. What industries benefit the most from domain-specific GenAI?
Industries that require high accuracy, specialization, and contextually relevant outputs benefit the most from domain-specific GenAI. Key industries include:
- Healthcare: For diagnostics, personalized treatment plans, and medical research.
- Finance: For risk assessments, fraud detection, and investment strategies.
- Legal: For case law analysis, legal documentation drafting, and contract reviews.
- Manufacturing: For optimizing production processes, predictive maintenance, and supply chain management.
10. How can domain-specific GenAI give my business a competitive advantage?
By integrating domain-specific data into your GenAI models, your business can:
- Generate more relevant and accurate insights tailored to your industry.
- Provide personalized customer experiences that stand out from competitors.
- Improve operational efficiency by automating tasks that require specialized knowledge.
- Make more informed decisions based on data-driven insights that align with your business needs, giving you an edge over businesses relying on generic AI models.
Take the Next Step Toward Enhanced AI Value
Integrating domain-specific data into GenAI models allows businesses to unlock deeper insights, improve accuracy, and gain a competitive edge. By focusing on data that is relevant to your industry, you can optimize your AI strategy and deliver more personalized, actionable results.
Start optimizing your AI strategy today by identifying and integrating the right domain-specific data. Doing so will ensure that your GenAI investments are delivering maximum value and driving meaningful business outcomes.