The Power of AI in Fraud Detection: Techniques, Challenges, and Strategies
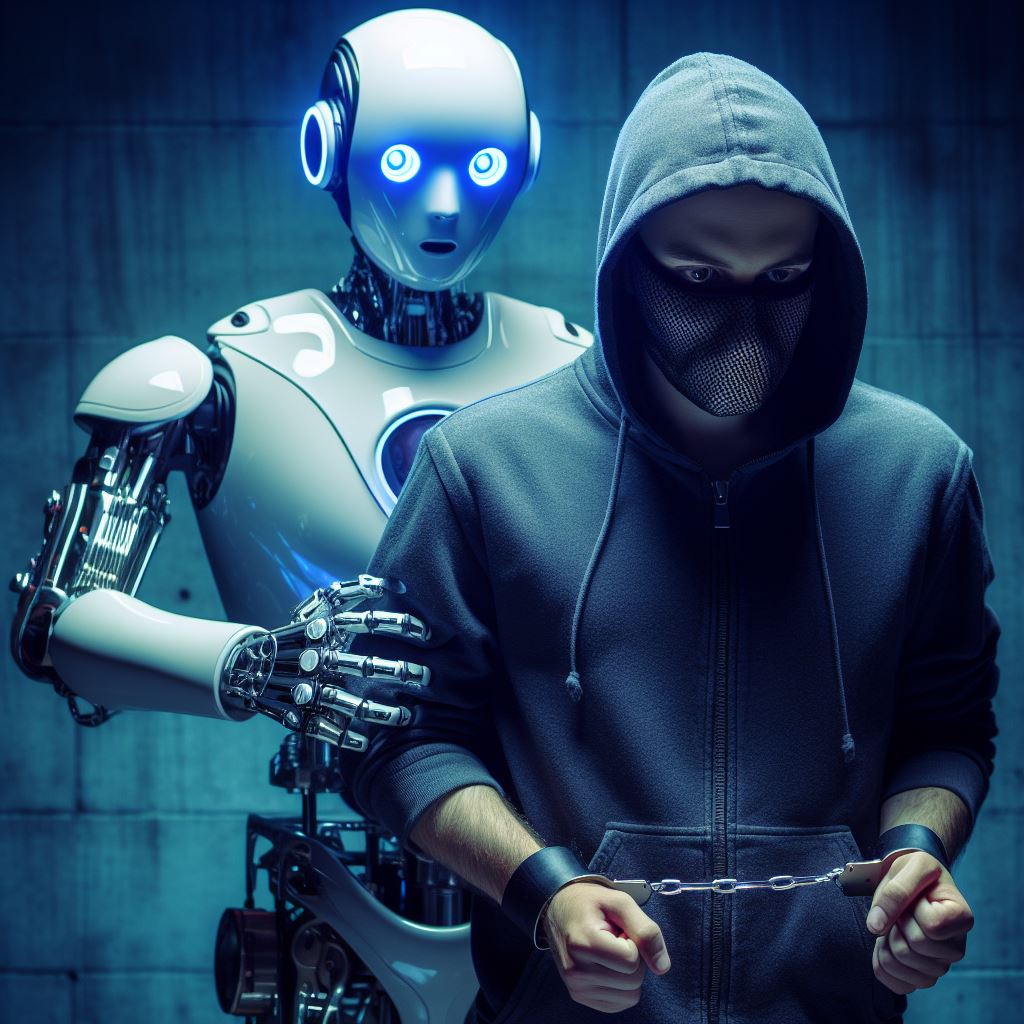
AI in Fraud Detection: Techniques and Challenges
AI for Fraud detection has always been a cat-and-mouse game, with fraudsters continually evolving their tactics and businesses improving their defenses. Today, artificial intelligence (AI) has become a formidable ally in combating fraud across multiple sectors including finance, healthcare, and online retail, thanks to its ability to learn from data and identify patterns, revolutionizing how organizations prevent fraud.
The impact of AI is particularly significant in the financial sector; a report on AI’s impact in the financial sector is evidence as the Fraud Detection and Prevention Market is projected to grow from USD 47.89 billion in 2024 to USD 126.69 billion by 2029, with firms enhancing fraud defenses through machine learning and behavioral analytics to tackle increasing and sophisticated fraud challenges. This growth is largely fueled by the burgeoning adoption of AI and machine learning technologies, which excel at analyzing vast datasets quickly and efficiently—far surpassing human capabilities.
The Concept of AI in Combating Fraud
AI for Fraud detection involves the use of machine learning algorithms, data analysis, and predictive modeling to identify and prevent fraudulent activities in real time. The core of AI’s effectiveness lies in its ability to learn from vast amounts of data and identify patterns that might be indicative of fraud. Unlike traditional methods, which often rely on static rules or manually created thresholds, AI systems continuously learn and adapt, becoming more proficient as they process more transactions.
AI for Fraud detection not only spots known fraud schemes but is also adept at detecting new patterns that may not have been previously identified. By leveraging technologies such as neural networks, decision trees, and clustering techniques, AI systems can analyze various data points like transaction amounts, frequencies, user behavior, and network data to make informed decisions about the legitimacy of a transaction or an activity.
How AI Improves upon Traditional Fraud Detection Methods
Traditional fraud detection methods typically rely on rule-based systems that flag transactions based on pre-set criteria. While effective to an extent, these systems can generate a high number of false positives and are often limited in their ability to adapt to new or evolving fraud tactics.
AI for Fraud detection systems offer several improvements over these traditional methods:
- Reduced False Positives: AI can discern subtleties and variations in data, reducing the likelihood of falsely flagging legitimate transactions as fraudulent, which can enhance user experience and operational efficiency.
- Dynamic Learning: Unlike static rule-based systems, AI models learn and evolve from each transaction, adapting to new patterns of fraudulent behavior that may not have been programmed into traditional systems.
- Speed and Efficiency: AI can process and analyze data at a scale and speed unattainable for human analysts, allowing for real-time fraud detection, which is critical in preventing fraud before it causes significant damage.
- Holistic Analysis: By integrating data from multiple sources and channels, AI provides a holistic view of a customer’s activities, enabling more accurate fraud detection by understanding broader behavioral patterns.
Overall, AI-driven systems are transforming fraud detection by offering smarter, more efficient, and adaptive solutions that are essential in today’s rapidly evolving digital environment.
Key Threats in AI Fraud Detection
AI technology faces complex hurdles that change in tandem with its increasing integration into fraud detection tactics. Scammers always change their tactics, using more intricate and recent strategies to elude detection systems that are set in stone. To ensure strong defensive mechanisms against fraud in a variety of areas, this section explores the numerous types of fraud that AI is entrusted with detecting and highlights the new dangers that AI must constantly adapt to combat.
Types of Fraud AI is Used to Detect
The most direct consequence of fraud is financial loss. According to a report by Deloitte, businesses lose an average of 5% of their annual revenue to fraud. This translates to significant amounts across different scales of businesses, impacting their profitability and sustainability. Early detection of fraud can significantly mitigate these losses.
Artificial Intelligence is employed across a broad spectrum of fraud AI for Fraud detection scenarios, each presenting unique challenges and requiring specialized approaches. Some of the primary types of fraud that AI is used to detect include:
- Financial Fraud: This includes everything from credit card fraud and wire fraud to complex financial crimes involving the manipulation of markets or large-scale corporate fraud. AI systems analyze transaction patterns, unusual account behavior, and other red flags to identify potential fraudulent activities.
- Identity Theft: AI helps in detecting identity fraud by analyzing behavioral patterns and cross-referencing data against known fraud indicators. This might include abnormal account activity or discrepancies in user-provided data compared to historical patterns.
- Insurance Fraud: From exaggerated claims to entirely fabricated accidents, AI tools scrutinize claims data against historical claims and external data sources to identify signs of fraud.
- Healthcare Fraud: AI detects irregular patterns in billing, unusual patient-provider interactions, and outlier claims that could indicate fraudulent activities, such as billing for services not rendered or overbilling for supplied services.
Emerging Threats and the Evolution of Fraud
As technology advances, so does the sophistication of fraud tactics, presenting ongoing challenges for AI in fraud detection:
- Synthetic Identity Fraud: Fraudsters create entirely new identities using a combination of real and fabricated information, or entirely fictitious data, making it one of the hardest frauds to detect. AI helps by identifying inconsistencies and patterns that do not match typical user behavior.
- Deepfake Technology: The use of AI-generated audio and video clips to impersonate legitimate users is a growing concern. AI-based systems are being trained to detect the subtle signs that content might be synthetically generated.
- AI-Powered Phishing Attacks: These attacks use AI to craft and send personalized messages that are more convincing, and thus harder to detect using traditional methods. AI systems counter these by analyzing communication for abnormal patterns and using natural language processing to detect phishing attempts.
The continuous evolution of fraud requires equally evolved AI solutions that not only detect known fraud types but also adapt to new threats as they emerge. This ongoing battle against fraud necessitates constant updates to AI models, incorporating the latest data and trends to stay ahead of fraudsters.
Data Handling for AI Fraud Detection
Effective data handling is a cornerstone of AI-driven fraud detection systems. The quality, accuracy, and integrity of the data not only influence the outcome of fraud detection but also define the reliability of the AI models used. As AI technologies strive to provide precise and actionable insights into fraud prevention, the role of meticulous data management becomes paramount. Here we discuss the critical importance of maintaining high data quality and integrity, followed by a discussion on the sophisticated techniques employed in data collection and preprocessing that prepare the ground for AI to function optimally in detecting fraudulent activities.
Importance of Data Quality and Integrity
In AI for Fraud detection, data is not just fuel—it is foundational. The accuracy, completeness, and integrity of data directly impact the effectiveness of AI models in detecting and preventing fraudulent activities. High-quality data ensures that AI systems can learn the most relevant patterns and anomalies indicative of fraud without being misled by errors or inconsistencies. Maintaining data integrity involves safeguarding data from unauthorized access or corruption throughout its lifecycle, which sustains trust in AI decisions and ensures compliance with regulatory standards such as GDPR and HIPAA.
Techniques for Data Collection and Preprocessing
The effectiveness of AI for Fraud detection starts with robust data collection and preprocessing techniques. These initial steps set the stage for the performance of subsequent analyses:
- Data Collection: Gathering data from diverse sources is vital to provide AI systems with a comprehensive view of user behavior and transactional patterns. This includes data from internal systems like transaction logs, account information, and external sources such as credit reports and public databases.
- Data Preprocessing: Before feeding it into AI models, data must be cleaned and formatted appropriately. Preprocessing tasks include:
- Handling Missing Data: Techniques such as imputation (filling missing values based on other data points) or using algorithms that support missing data are employed to ensure model accuracy isn’t compromised.
- Normalization: Scaling data to a standard range or format, which is important for models that are sensitive to the scale of input data, such as neural networks.
- Feature Engineering: Extracting and selecting those features that are most predictive of fraud. This might include deriving new variables from existing data, such as calculating the frequency of transactions in a given period.
- Data Balancing: Addressing issues with unbalanced datasets where fraudulent transactions are much rarer than legitimate ones. Techniques like oversampling the minority class or undersampling the majority class can help balance the data.
These preprocessing steps are critical for maximizing the predictive power of AI models, enabling them to detect subtle patterns and signals of fraudulent activities effectively.
Model Training for Fraud Detection
Model training is a critical phase in the development of AI for Fraud detection systems, involving the creation of algorithms that can learn from and make predictions on data. This process begins with data collection and preprocessing, as discussed earlier, followed by the selection of an appropriate model type based on the specific needs of the fraud detection task. The training involves feeding the model large volumes of historical data—both fraudulent and legitimate transactions—so that it can learn to differentiate between the two. The model’s performance is continuously evaluated and refined using a subset of the data, known as the validation set, to ensure it generalizes well to new, unseen data before it’s finally deployed in real-world settings.
Common Algorithms and Techniques Used in Fraud Detection Models
Several algorithms and techniques are particularly suited for fraud detection, each with its strengths:
- Decision Trees: These are straightforward and effective for rules-based scenarios where decisions follow a hierarchical model.
- Random Forests: An ensemble of decision trees, this method is more robust and less prone to overfitting, making it ideal for handling complex datasets with many variables.
- Neural Networks: Especially deep learning models, which are powerful in detecting non-linear relationships and interactions in high-dimensional data.
- Support Vector Machines (SVM): Known for their effectiveness in classification tasks, SVMs can be particularly useful in distinguishing between fraudulent and non-fraudulent transactions.
- Anomaly Detection Techniques: These are critical in identifying rare events or observations that raise suspicions of fraud.
Challenges in Training AI Models for Fraud Detection
Training AI for fraud detection is filled with challenges that can affect their efficiency and accuracy:
- Imbalanced Data: Fraudulent transactions are typically much rarer than legitimate ones. This imbalance can cause models to be biased towards predicting the majority class, often ignoring the minority fraud class.
- Evolving Fraud Tactics: Fraudsters continuously refine their strategies to evade detection. AI models must be dynamic, requiring frequent updates and retraining to keep pace with new fraud tactics.
- Feature Selection: Determining which features are most predictive of fraud can be complex, requiring deep domain knowledge and continuous experimentation.
- Model Overfitting: There is a risk that a model learns the training data too well, including the noise and anomalies, which can degrade its performance on new, unseen data.
- Regulatory Compliance: Models must not only be effective but also compliant with legal standards, ensuring that they do not inadvertently discriminate or violate privacy laws.
Model Maintenance and Upkeep
AI models for fraud detection are not set-and-forget systems; they require ongoing maintenance to ensure their effectiveness over time. Continuous model evaluation and updating are important because the patterns of fraudulent activity continually evolve as fraudsters develop new methods to circumvent detection measures. Additionally, as transaction behaviors and trends shift over time, models that are not regularly updated can become less accurate and relevant. This ongoing maintenance ensures that the model remains sensitive to the latest fraud tactics and responsive to changes in normal transaction patterns.
Strategies for Maintaining Accuracy Over Time
AI models for fraud detection require consistent monitoring and updating to remain effective against evolving fraud tactics. As fraudulent strategies and transactional behaviors change, models that aren’t regularly updated risk becoming obsolete.
- Regular Re-training: Refresh the model periodically with the latest data, including new fraudulent activities and legitimate transactions.
- Model Monitoring: Keep track of performance metrics such as precision, recall, and accuracy to ensure the model remains effective.
- Feedback Loops: Integrate insights from human experts back into the system to refine and improve model predictions.
- Adaptive Algorithms: Use algorithms that automatically adjust to new trends without full retraining.
- Ensemble Techniques: Employ multiple models in tandem to enhance prediction accuracy and mitigate individual model weaknesses.
The long-term accuracy and reliability of AI-driven fraud detection systems involves a proactive approach to model maintenance. By regularly updating and refining these models, businesses can effectively adapt to the dynamic nature of fraud, maintaining robust defenses against new and emerging threats.