The Future of Claims Management: How AI is Revolutionizing Logistics
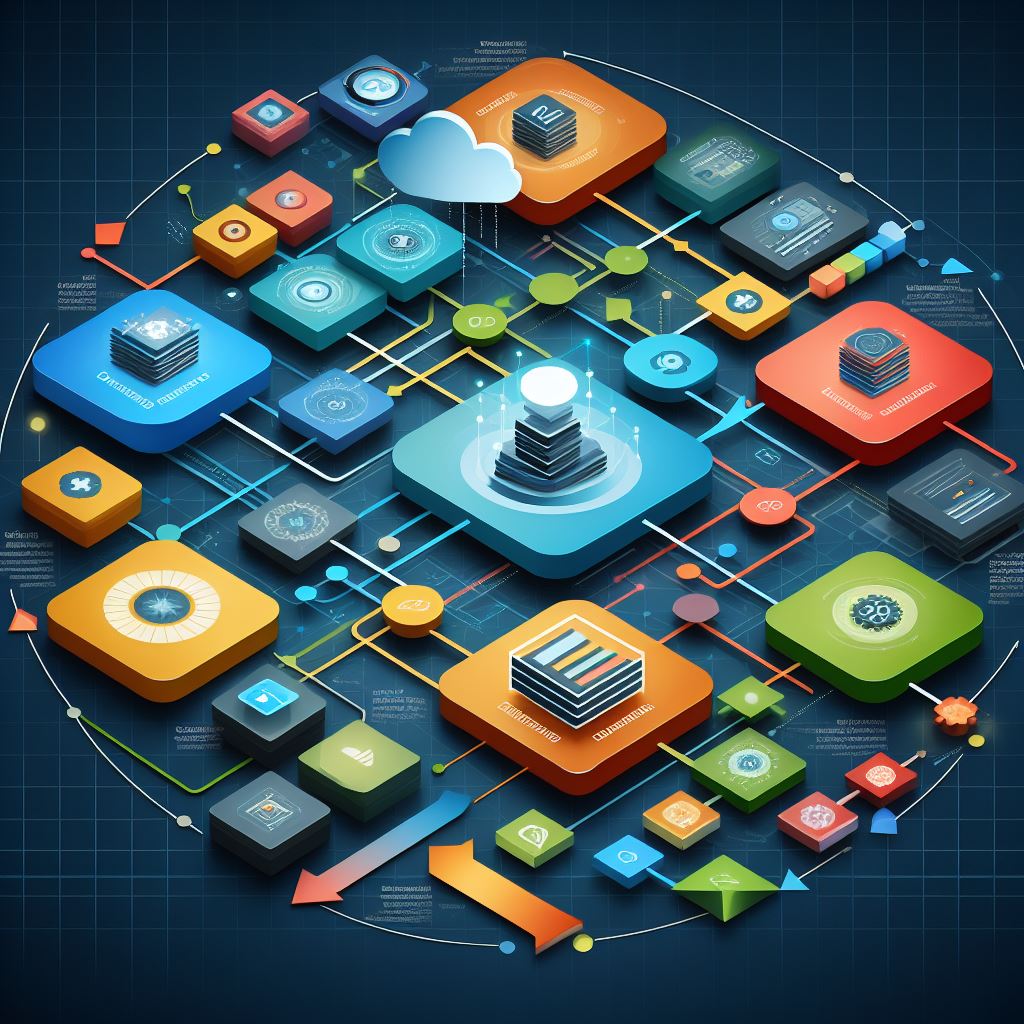
7 AI Strategies for Effective Claims Management in Logistics
The global economy relies on the logistics business to effectively get commodities from producers to consumers. However, logistics businesses struggle with claims management. Claims management may be complicated and time-consuming, involving damaged items, late delivery, and billing disputes.
Using paper paperwork and human decision-making, logistics claims management has traditionally been laborious. This inefficient and error-prone method slows claim processing and dissatisfies clients.
Claims processing is just one area where artificial intelligence has reduced logistical costs by 15%, according to a McKinsey analysis that quantifies the effect. Accenture found 36% of companies using AI for logistics had positive results.
The logistics industry must immediately adopt AI claims management technologies. Claims administration might be automated and optimized with the use of artificial intelligence technologies such as machine learning, natural language processing, and predictive analytics, which could lead to faster resolution times, lower costs, and happier customers.
This article will discuss seven AI tactics that may assist logistics organizations handle claims, including their advantages and real-world examples. Logistics firms may improve claims handling and gain a competitive advantage by using AI.
1. AI-Powered Data Collection
AI technology like IoT sensors and automated data entry systems help logistics companies gather and handle claims data. These technologies allow logistics organizations to collect real-time cargo data, including location, temperature, and condition, to authenticate claims and discover concerns early.
IoT sensors are embedded in various assets, including vehicles, containers, and packages, allowing logistics companies to track their movements and monitor environmental conditions. These sensors collect data such as GPS coordinates, temperature, humidity, and shock levels, providing valuable insights into the condition of the goods in transit.
Automated data entry systems, on the other hand, streamline the process of collecting and entering data into claims management systems. These systems can automatically capture data from documents such as bills of lading, delivery receipts, and inspection reports, reducing the need for manual data entry and minimizing errors.
One of the key benefits of real-time data collection is its ability to identify and address potential claims issues promptly. By continuously monitoring shipments and collecting data in real-time, logistics companies can detect deviations from expected norms, such as delays, route deviations, or temperature excursions, which could indicate potential claims situations.
For example, if an IoT sensor detects that a shipment of perishable goods has been exposed to temperatures outside the acceptable range, it can trigger an alert to the logistics company, allowing them to take corrective action, such as rerouting the shipment or replacing the goods, before a claim is filed.
2. Predictive Analytics for Risk Assessment
Predictive analytics, a subset of AI, is increasingly being used in the logistics industry to analyze historical data and predict potential risks in operations. By analyzing past trends and patterns, predictive analytics can help logistics companies identify potential issues before they occur, allowing them to take proactive measures to mitigate risks and reduce the likelihood of claims.
One way AI can be used for predictive analytics in logistics is by analyzing historical shipping data to identify patterns that may indicate potential risks. For example, AI algorithms can analyze past delivery routes, weather and traffic patterns to predict the likelihood of delays or accidents on specific routes. By identifying high-risk routes or times of year, logistics companies can take proactive measures to minimize the impact of these risks, such as rerouting shipments or adjusting delivery schedules.
Another use of predictive analytics in logistics is in predicting equipment failures. By analyzing data from IoT sensors and maintenance records, AI algorithms can predict when a vehicle or piece of equipment is likely to fail, allowing for proactive maintenance to be performed before a breakdown occurs. This not only reduces the risk of delays but also helps to extend the life of equipment, reducing overall maintenance costs.
Case studies and examples can help illustrate the effectiveness of predictive analytics in reducing claims in logistics. For example, a study by a major logistics company found that by using predictive analytics to identify high-risk shipments and take proactive measures to mitigate risks, they were able to reduce claims by 20% over one year.
3. Automated Claims Processing
Automated claims processing is a game-changer in the logistics industry, offering a streamlined approach to handling claims from submission to settlement. AI-powered systems can automate various stages of the claims processing workflow, including claim submission, validation, and settlement. These systems use algorithms to analyze claim data, verify policy details, and process payments, reducing the need for manual intervention.
Advantages of Automation
- Speed: Automated claims processing is much faster than manual processing, leading to quicker resolution times and improved customer satisfaction. Tasks that might take days or weeks to complete manually can be done in minutes with AI.
- Accuracy: Automation leads to increased accuracy in claims processing. AI systems are less prone to errors than humans, reducing the risk of costly mistakes. By automating repetitive tasks, such as data entry and verification, AI ensures that claims are processed correctly the first time.
- Cost Savings: Automation can lead to significant cost savings for logistics companies. By reducing the need for manual labor, AI helps companies save on staffing costs. Additionally, by streamlining the claims processing workflow, AI can help companies reduce operational costs and improve efficiency.
- Improved Customer Experience: Automation improves the customer experience by reducing the time it takes to process requests. Customers appreciate fast and efficient service, and automated claims processing delivers on this expectation.
Case Study
For example, a logistics company implemented an AI-powered claims processing system and saw a 30% reduction in claims processing time and a 15% reduction in errors. This led to increased customer satisfaction and significant cost savings for the company.
Overall, automated claims processing is transforming the way logistics companies manage claims, offering a faster, more accurate, and cost-effective solution. By leveraging AI technologies, companies can streamline their claims-processing workflow and deliver a better experience for both customers and employees.
4. Natural Language Processing for Customer Communication
In the logistics industry, effective customer communication is essential, especially during the claims process. Natural Language Processing (NLP) powered chatbots and virtual assistants are transforming customer communication by providing real-time updates and resolving queries efficiently. This section explores how AI-driven NLP technologies are transforming customer communication in claims management.
AI-driven Chatbots and Virtual Assistants
- Real-time Updates: NLP-powered chatbots can provide customers with real-time updates on their claims, including status updates, estimated resolution times, and next steps. This helps keep customers informed and reduces the need for them to follow up manually.
- Efficient Query Resolution: NLP enables chatbots to understand and respond to customer queries in natural language, making interactions more conversational and efficient. Chatbots can provide immediate responses to common queries, freeing up human agents to focus on more complex issues.
Benefits of NLP in Customer Communication
- Improved Customer Experience: NLP-powered chatbots provide a more seamless and responsive customer experience by offering real-time updates and resolving queries efficiently. This leads to higher customer satisfaction and loyalty.
- Cost Savings: By automating customer communication, NLP-powered chatbots can help reduce the need for human agents, leading to cost savings for logistics companies.
- Scalability: Chatbots can handle a large volume of customer queries simultaneously, making them ideal for managing peak periods or sudden spikes in claims.
NLP-powered chatbots and virtual assistants are transforming customer communication in the logistics industry, offering real-time updates and efficient query resolution. By leveraging these technologies, logistics companies can improve the customer experience, reduce costs, and scale their customer communication efforts effectively.
5. Fraud Detection and Prevention
Fraudulent claims pose a significant challenge for logistics companies, leading to financial losses and reputational damage. Artificial Intelligence (AI) has emerged as a powerful tool for detecting and preventing fraud in the logistics industry. This section explores the role of AI in fraud detection and prevention, highlighting key algorithms and technologies used for this purpose.
Role of AI in Fraud Detection and Prevention
Data Analysis: AI algorithms can analyze large volumes of data to identify patterns and anomalies that may indicate fraudulent activity. By analyzing historical claims data, AI can identify trends and patterns associated with fraudulent claims.
Behavioral Analysis: AI can also analyze the behavior of users to detect signs of fraud. For example, AI algorithms can analyze user interactions with digital platforms to identify suspicious behavior, such as multiple claims being filed from the same IP address.
Real-time Monitoring: AI-powered systems can monitor transactions in real-time to detect and prevent fraudulent activity as it occurs. Using machine learning algorithms, these systems can learn from previous data to improve their detection capabilities over time.
Examples of AI Algorithms and Technologies
Anomaly Detection: Anomaly detection algorithms are used to identify patterns in data that deviate from expected norms. In the context of fraud detection, anomaly detection can be used to identify unusual patterns in claims data, such as unusually high claim amounts or a high frequency of claims from a particular region.
Pattern Recognition: Pattern recognition algorithms are used to identify recurring patterns in data. In fraud detection, pattern recognition can be used to identify common fraud schemes, such as false billing or collusion between employees and customers.
Machine Learning: Machine learning algorithms, like supervised and unsupervised learning, are used to train AI systems to detect fraud. These algorithms can learn from previous data to identify new and emerging fraud patterns.
In the logistics business, AI is vital for finding and avoiding false claims. Logistics firms may enhance their fraud detection skills, decrease financial losses, and safeguard their brand by using AI algorithms and technology.
6. Continuous Improvement through Machine Learning
Machine learning (ML) algorithms can analyze claims data to identify patterns and trends, providing valuable insights for the continuous improvement of claims management processes. These algorithms can process large volumes of data quickly and efficiently, identifying patterns that may not be apparent to human analysts. By analyzing historical claims data, ML algorithms can identify trends in claims frequency, severity, and root causes, helping companies optimize their claims management processes.
Benefits of Using ML for Continuous Improvement:
Using ML for continuous improvement offers several benefits. Firstly, ML algorithms can help companies identify and address inefficiencies in their claims management processes, leading to cost savings and improved operational efficiency. Secondly, ML enables companies to proactively identify and mitigate risks, reducing the likelihood of costly claims. Thirdly, by continuously analyzing data and adapting to new information, ML helps companies stay agile and responsive to changing market conditions, improving their overall competitiveness.
7. Dynamic Resource Allocation
AI can optimize resource allocation for claims management by analyzing real-time data on claim volume, complexity, and urgency. By continuously analyzing incoming claims data, AI algorithms can dynamically assign resources such as personnel, equipment, and funds to where they are most needed. This ensures that claims are processed efficiently and promptly, reducing delays and improving customer satisfaction.
One of the key benefits of dynamic resource allocation is its ability to adapt to changing conditions. For example, during periods of high claim volume, AI can allocate additional resources to ensure that claims are processed quickly. Conversely, during periods of low claim volume, AI can reallocate resources to other areas of the business, reducing costs and improving overall efficiency.
Dynamic resource allocation also helps companies manage their resources. By analyzing data on resource utilization and performance, AI can identify opportunities for improvement and optimization. For example, AI may identify bottlenecks in the claims processing workflow and recommend changes to streamline operations.
Conclusion
Artificial Intelligence (AI) has changed claims management in the logistics industry, offering unparalleled accuracy, efficiency, and customer satisfaction. From streamlining processes and reducing costs to enhancing decision-making and improving compliance, AI is transforming the way logistics companies handle claims. As AI continues to evolve, its role in claims management will only become more significant, helping companies stay competitive in an ever-changing landscape.Â