An In-Depth Guide to Generative AI in Manufacturing for 2024
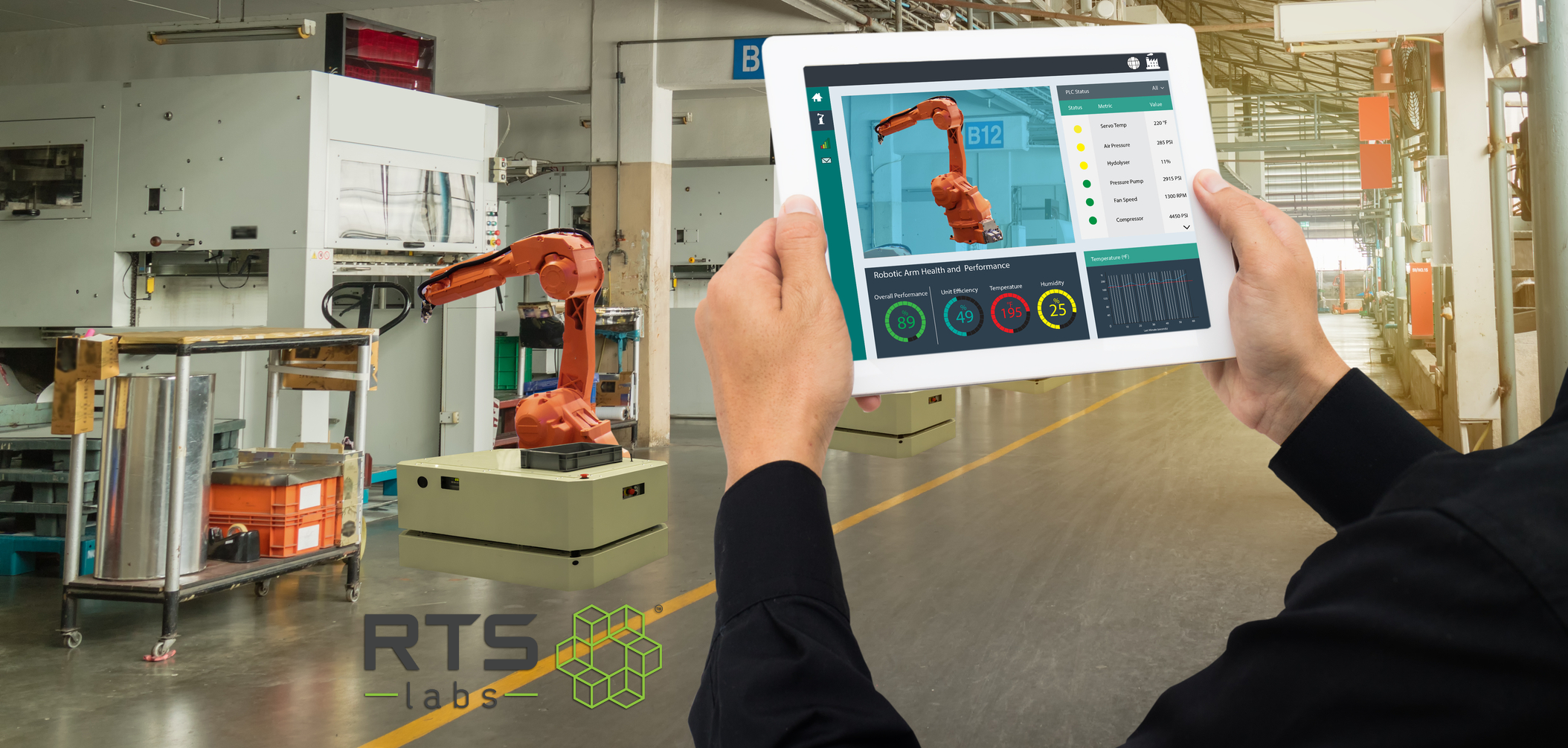
Generative AI is changing how we think about manufacturing. Unlike traditional AI, which follows set rules, generative AI uses data to create new ideas and designs. This isn’t a minor update, it’s a big shift in how products can be made. Think of it as giving machines the ability to suggest new ways to design or produce things, not just follow a script.
In 2024, generative AI is more important than ever. Why? Because it helps manufacturers keep up in a market where the demand for innovation is constant. With generative AI, companies can create prototypes faster, make products more customized to customer needs, and streamline their supply chains. The trend is clear: manufacturers need to understand and use these new AI technologies if they want to stay competitive.
Understanding Generative AI
If you have ever wondered what’s behind the buzz around generative AI, you are not alone. This technology is doing more than just making headlines; it’s fundamentally changing how industries operate, especially manufacturing. But before we go into the specifics of its impact on production lines and supply chains, it’s important to understand what generative AI really is, how it works, and why it’s different from the AI you might already know. By grasping these basics, you will see why this isn’t just another tech trend but a tool for innovation and efficiency.
What is Generative AI?
Generative AI is artificial intelligence that creates new content, such as text, images, music, or product designs, instead of just analyzing existing data. Unlike traditional AI, which follows predefined rules or relies on past data to make decisions, generative AI uses algorithms to generate unique and creative outputs.
For example, it can design a new product by exploring countless design possibilities, compose original music by understanding patterns in existing compositions, or create digital art based on a few descriptive prompts. This ability to generate rather than replicate makes generative AI particularly powerful and versatile across various fields.
Core Components of Generative AI
To grasp how generative AI is helping industries like manufacturing, it’s important to break down what makes it tick. Generative AI involves several key components working together to produce innovative outcomes. Let’s explore these core elements, machine learning models, data requirements, and specific algorithms, each of which allows AI to generate new and useful outputs.
- Machine Learning Models
Generative AI relies on machine learning models, which serve as the core “brains” of the technology. These models are trained to recognize patterns in large datasets. Unlike traditional AI, which may only analyze data to make predictions, generative AI uses these patterns to create something entirely new. For example, in manufacturing, a generative AI model can examine thousands of product designs and then generate a completely new design that is more efficient or cost-effective. This capability allows generative AI to innovate beyond just reproducing existing knowledge, enabling it to create original outputs based on what it has learned.
- Data Requirements
Generative AI relies heavily on large amounts of diverse data to function effectively. The more data these models have, the better they can learn and identify patterns and subtle nuances. In manufacturing, this means providing AI with comprehensive data from every stage of the production process or every product design. This extensive data helps the AI develop a deep understanding, enabling it to generate innovative solutions and create new designs or processes that are more efficient and effective. The richer and more varied the data, the more capable the AI becomes in producing unique and useful outputs.
- Algorithms and Techniques
The power of generative AI lies in its algorithms and techniques, which allow it to create new and innovative outputs. One widely used method is Generative Adversarial Networks (GANs). GANs consist of two models that work together in a competitive process: one model generates new data (such as a product design). In contrast, the other model evaluates the data to determine if it’s real or generated.
This “adversarial” setup helps both models improve over time; the generator gets better at creating realistic data, and the evaluator becomes more skilled at detecting what’s generated. This iterative learning process enables generative AI to continuously enhance its capabilities, making it a powerful tool for creating unique solutions.
Applications of Generative AI in Manufacturing
Generative AI in manufacturing goes beyond a simple tech upgrade; it fundamentally changes how processes are managed. By using advanced AI methods, manufacturers can enhance various aspects of their operations, such as product design, quality control, and supply chain optimization. This technology helps create more efficient workflows and higher-quality products.
The key areas where generative AI is making a noticeable impact are discussed below:
Product Design and Prototyping
In manufacturing, product design and prototyping have traditionally been time-consuming and resource-intensive processes. Generative AI offers a new approach by automating parts of the design phase and providing creative suggestions based on data. This leads to faster prototyping cycles and opens up possibilities for designs that are both novel and optimized for performance. In 2024, examples of AI-driven prototypes are becoming more common, showcasing the potential for more dynamic and adaptive design processes.
Supply Chain Optimization
Supply chains are the arteries of manufacturing, and optimizing them can lead to huge gains in efficiency and cost savings. Generative AI is stepping in by analyzing vast amounts of supply chain data to find patterns and predict potential bottlenecks or disruptions. This proactive use of AI allows companies to better manage their resources, reduce waste, and improve overall supply chain resilience.
Predictive Maintenance
Maintenance has always been reactive, often triggered by equipment failure or scheduled checks. With generative AI, this changes to a more predictive model. By continuously analyzing data from machines, AI can forecast maintenance needs before a breakdown occurs, preventing costly downtime and extending the lifespan of equipment. This shift from reactive to predictive maintenance is becoming increasingly popular as companies recognize its cost and efficiency benefits.
Quality Control and Defect Detection
Quality control is a critical aspect of manufacturing, and even minor defects can lead to significant losses. Generative AI enhances this process by using advanced algorithms to detect defects that the human eye might miss. It also increases accuracy and speeds up the inspection process so that only the best products make it to market. AI-driven quality control methods are setting new standards for efficiency and reliability in product manufacturing.
Benefits of Generative AI in Manufacturing
Generative AI is changing manufacturing by fostering new ways to innovate, reduce costs, accelerate processes, and enhance decision-making. It helps manufacturers create smarter, more efficient workflows and develop higher-quality products with greater precision. Let’s examine the specific advantages generative AI offers to the manufacturing industry.
Enhanced Innovation
Generative AI allows manufacturers to push the boundaries of what’s possible. By analyzing vast datasets and exploring a range of possibilities, AI can propose innovative designs that humans might never consider. This capability leads to more creative products and gives companies a significant edge over competitors relying solely on traditional design methods.
Cost Efficiency
Cost management is crucial in manufacturing, and generative AI offers a powerful tool for optimizing expenses. By streamlining production processes and minimizing waste, AI helps manufacturers reduce costs significantly. Whether through smarter material use or more efficient workflow, the impact on the bottom line can be substantial.
Faster Time to Market
In a competitive market, speed is often as important as quality. Generative AI accelerates the product development cycle by automating and enhancing various stages of production. This means that products can move from concept to market faster, allowing companies to capitalize on new trends and customer demands more swiftly.
Improved Decision-Making
Manufacturers face countless decisions daily, from production schedules to quality checks. Generative AI helps simplify these decisions by providing accurate and actionable data-driven insights. This support enables manufacturers to make better, more informed choices that can improve overall efficiency and output quality.
Challenges and Considerations in Implementing Generative AI
Adopting generative AI in manufacturing brings significant challenges that companies must address to maximize its benefits. Key challenges include data privacy and security, integration with legacy systems, skill shortages, and high costs. Effectively managing these issues is crucial for a successful AI deployment.
Generative AI relies on large datasets, often containing sensitive information, increasing the risk of data breaches and cyber threats. To mitigate these risks, companies should use privacy-enhancing technologies (PETs) like data anonymization and encryption and strengthen cybersecurity measures such as zero-trust architectures.
- Adopt Privacy-Enhancing Technologies (PETs): Techniques like anonymization and encryption protect sensitive data before processing, minimizing exposure risks.
- Strengthen Cybersecurity Frameworks: Implement zero trust models to continuously verify users and devices, securing AI systems against threats.
Integrating AI with existing systems can be challenging due to compatibility issues with older equipment and software.
- Use Modular AI Solutions: Gradual integration of AI into specific processes can reduce disruptions.
- Invest in Middleware: Middleware bridges gaps between legacy systems and AI technologies, ensuring smooth integration.
By addressing these challenges with targeted strategies, manufacturers can enhance productivity, reduce costs, and maintain competitiveness in a rapidly changing industry.
Future Trends in Generative AI for Manufacturing
Imagine being able to design highly customized products on the fly, reduce waste dramatically, and even revolutionize entire production methods. These aren’t just distant possibilities, but the emerging trends shaping the industry today. Companies are now leveraging generative AI to create more personalized manufacturing processes, drive sustainability, and push the boundaries of what’s possible with additive manufacturing.
AI-Driven Customization
Generative AI is paving the way for mass customization in manufacturing, allowing companies to offer personalized products without sacrificing efficiency. This technology enables manufacturers to rapidly adapt designs and production processes, responding to individual customer preferences in real-time. For instance, AI-driven customization leverages data to tailor products at scale, making it possible to offer unique features or design elements without increasing production costs.Â
Predictions suggest that as AI continues to evolve, we will see even more sophisticated levels of personalization, allowing manufacturers to customize aesthetics, functionality, and performance to meet specific customer needs. This trend is set to transform industries such as consumer electronics, automotive, and fashion, where personalization is becoming a key differentiator in the market.
Sustainable Manufacturing with AI
Generative AI is also contributing significantly to sustainable manufacturing practices. By optimizing design and production processes, AI helps reduce waste and improve resource efficiency. For example, generative AI can simulate various production scenarios to determine the most resource-efficient methods, thus minimizing the environmental footprint of manufacturing activities. This includes optimizing material usage, reducing energy consumption, and reducing emissions.
AI-driven tools can assist in designing products that are easier to recycle or have a reduced carbon footprint from the outset. As sustainability becomes a crucial business objective, AI’s role in promoting eco-friendly practices will only expand, helping companies meet both regulatory requirements and consumer expectations for greener products.
AI in Additive Manufacturing
Integrating generative AI with additive manufacturing (3D printing) is unlocking new possibilities for creating complex and customized components that were previously difficult or impossible to produce. Generative AI can optimize the design process for additive manufacturing by suggesting the best materials, structures, and printing methods to achieve desired properties, such as strength, flexibility, or weight reduction. This synergy allows for rapid prototyping and production of parts with enhanced performance characteristics, which can be iteratively improved based on real-time data and feedback.
The future of additive manufacturing looks bright as AI continues enhancing its capabilities, offering more innovative solutions and expanding the range of applications in aerospace, healthcare, and automotive industries.
Case Studies of Generative AI in Manufacturing
Real-world applications of generative AI in manufacturing provide a window into the technology’s transformative potential. By examining specific case studies, we can see how companies leverage AI to innovate, improve efficiency, and reduce costs. These examples highlight the successes and challenges faced when integrating AI into complex industrial environments.
Here are a few key examples to help you understand how generative AI is used today and what lessons can be learned for future implementations.
Case Study 1: Bosch Digital Twin Industries
Bosch has implemented generative AI in its digital twin technology to optimize asset performance through predictive maintenance and enhanced decision-making. By leveraging multi-sensor data and AI algorithms, Bosch’s system provides maintenance engineers with actionable insights to prevent equipment failures and optimize operations.
The use of generative AI chatbots in this context helps retrieve and analyze relevant information quickly, facilitating faster troubleshooting and maintenance processes. This approach not only improves equipment uptime and reduces costs but also enhances the overall efficiency of the manufacturing process.
Case Study 2: Tulip and AWS Collaboration
In collaboration with AWS, Tulip has developed a platform that integrates generative AI to augment frontline operations in manufacturing. This platform uses AI to automate documentation, provide contextualized instructions, and enhance knowledge sharing across the workforce. By leveraging services like Amazon Bedrock, Tulip’s platform helps streamline production processes, improve quality control, and boost workforce engagement.
The integration of generative AI in Tulip’s operations showcases the potential of AI to transform traditional manufacturing processes into more agile, data-driven workflows that enhance productivity and quality.
Key Takeaways from AI Implementations
From these case studies, it is clear that the successful implementation of generative AI in manufacturing hinges on several factors:
- Data Integration: Leveraging AI effectively requires a robust data infrastructure that integrates various data sources across the manufacturing process.
- Customization and Flexibility: AI applications that offer customization and can be adapted to specific needs provide significant competitive advantages.
- Training and Skill Development: Upskilling the workforce to effectively use AI tools is critical to maximizing the benefits of these technologies.
These insights and case studies illustrate the transformative potential of generative AI in manufacturing and provide a roadmap for organizations aiming to integrate this technology into their operations.
Conclusion
Generative AI is transforming manufacturing by driving innovation, enhancing cost efficiency, reducing time to market, and improving decision-making. However, integrating this technology isn’t without its challenges. Data privacy and security, system integration issues, skill gaps, and implementation costs are all hurdles that companies must navigate to fully capitalize on AI’s benefits. Despite these obstacles, the potential of generative AI in creating more customized, sustainable, and efficient manufacturing processes makes it a tool for the future.
As manufacturers look to 2024 and beyond, adopting AI-driven solutions can provide a significant competitive edge. Now is the time to explore how generative AI can fit into your strategic goals and operational needs. For those ready to lead in this new manufacturing era, RTS Labs offers expertise in leveraging advanced AI technologies to optimize your production processes and drive growth. Reach out to RTS Labs to discover how we can help you integrate generative AI into your business strategy for maximum impact.
FAQs
- What is generative AI in manufacturing?
Generative AI in manufacturing uses AI algorithms to create new designs and optimize processes, enhancing innovation and efficiency.
- How does generative AI improve manufacturing processes?
It optimizes product designs, reduces waste, speeds production, and supports predictive maintenance.
- What are the benefits of using generative AI in manufacturing?
The benefits include cost savings, faster production times, improved product quality, and better decision-making.
- What are the challenges of implementing generative AI in manufacturing?
Data privacy, system integration, skill gaps, and high costs are the challenges.
- How can manufacturers start using generative AI?
They can start by focusing on high-impact areas, integrating AI gradually, and investing in training.
Further Reading
The following resources delve deeper into how generative AI is transforming the manufacturing industry. From optimizing supply chains to revolutionizing factory operations, these articles provide valuable insights into the challenges AI addresses and its future potential in reshaping manufacturing processes.
- 4 ways generative AI addresses manufacturing challenges | IBM
- Harnessing generative AI in manufacturing and supply chains
- Generative AI’s Role in the Factory of the Future | BCG