4 Ways Data Science Is Transforming Sales and Marketing, Part 1
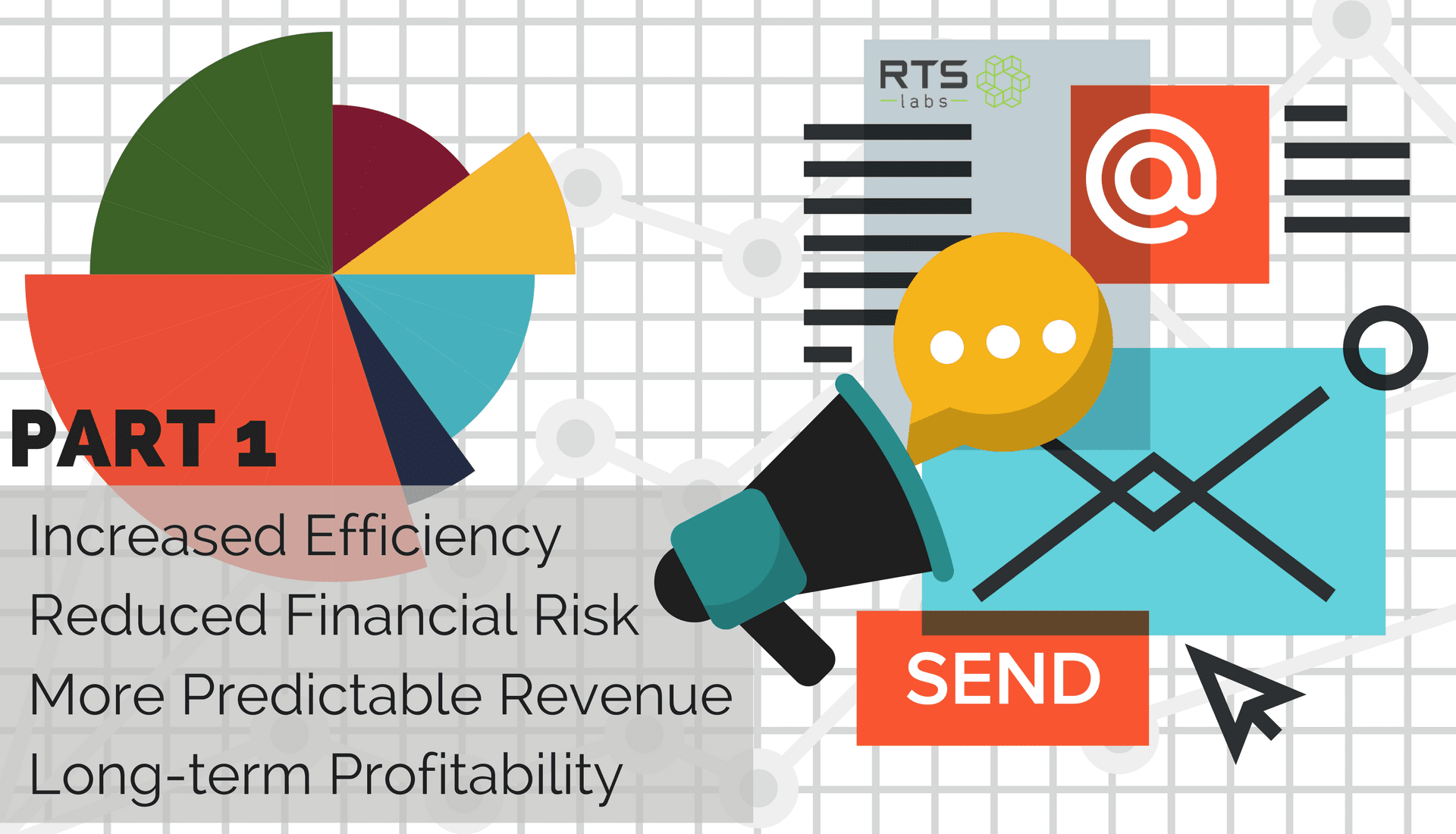
Sales and marketing as we know them are dying.
And not surprisingly, the use of digital marketing keeps growing. Soon everything we use will have a digital connection. In only a few years, some of the standard skills used in sales and marketing campaigns will be obsolete.
In their place will be vast amounts of data, and tools like data science, that give marketers capabilities they once only imagined.
But it takes true vision and determination to transform digitally and capitalize on this opportunity. Unless you, your CEO, and other C-Suite leaders have a massive commitment to using the firm’s resources effectively, it’ll never happen.
To keep marketers from getting bogged down, tools like data science will need to handle the details. That way, marketing leaders can focus on asking the big, important questions; such as how we can:
- Increase marketing efficiency
- Reduce our financial risk
- Make profit more predictable
- Increase our long-term revenue
If you’re looking for clarity on how to use data science to lead your organization into the future, take a look at these 4 ways data science is transforming sales and marketing to gain ideas and learn about best practices.
Increase Efficiency
The more you know about the people in your target markets, the better your marketing will be. And there’s plenty to know. Their needs, challenges, and interests all play a role in shaping their buying decisions.
With more content than ever for your audience to digest, your prospects are already overloaded with information. Yet, among the two quintillion bytes of data generated every day, your marketing message must stand out in the clutter.
For that to happen, your messages must be more personal, targeted, and relevant than ever before. The key to getting results is having the correct data about the people you’re trying to reach. However, customer profiling is a time- and labor-intensive process. That’s where data science can help.
One example of this newfound efficiency would be the lack of need for buyer personas. When used effectively, data science will eradicate the need to manually create buyer personas. Imagine having rich, real-time data that continually updates your customer profile information.
Then, instead of the typical trial-and-error approach to target marketing, you’ll be able to keep up with changes in customer demand through real-time intelligence. Data science will help you dig deeper into the numbers. Then, you’ll have a clearer idea of where you should spend your marketing budget to reach your most desirable audience and get the highest ROI.
Based on demographic, firmographic, behavioral, and contextual data, your marketing systems will be able to digest and act on the data based on your preset goals. The activities of your marketing and sales teams will easily integrate that data, too.
Reduce Financial Risk
Difficulty in calculating marketing ROI is one of the biggest frustrations for every professional digital marketer. That’s because digital marketing isn’t as tangible as other parts of a business. It’s a multidimensional process with many different channels.
Sometimes even the smallest detail can significantly affect a marketing ROI calculation. Yet, marketers must measure a multitouch customer journey that’s the sum of many diverse, often difficult to measure, interactions.
Another challenge is the cumulative nature of marketing. Marketing ROI may not show up for months or even years, so campaigns may be standing on the shoulders of earlier work.
The real problem with calculating marketing ROI is not that it’s impossible but that it’s difficult to do affordably. While marketing ROI may not be an exact science now, it could be with the help of data science.
To calculate marketing ROI more effectively, the key is to find and track which variables and outcomes are most relevant to your business. The following key performance indicators (KPIs), combined with data science, can help you calculate inbound marketing ROI:
- Landing page conversion rates
- Organic traffic
- Social media traffic and conversion rates
- Mobile traffic, leads, and conversion rates
- Traffic-to-lead ratio
- Lead-to-customer ratio
- Cost per lead
- Sales revenue
- Customer lifetime value
Your data-driven solution could increase the accuracy of your marketing ROI calculations by reviewing KPIs over time. For example, short-term marketing investments and sales growth would be better measured quarterly or annually. On the other hand, long-term brand investments would be better measured over a 2-3 year period or longer.
While improved target marketing and better ROI will boost marketing results, what about sales?
More Predictable Revenue
Sales forecasting is notoriously hard to do. In many cases, you’d have better luck tossing a coin. Few organizations are happy with the accuracy of their sales forecasts because they depend on gut feeling and countless spreadsheets.
The challenge is that salespeople have their own preconceived ideas about which leads will turn into sales. It’s not uncommon for sales management to spend months analyzing data and then not give their sales team enough time to act on it. These tail-chasing exercises are pointless anyway, if salespeople aren’t entering correct information to begin with.
On top of a lack of personal accountability from salespeople, displaying overconfidence or sandbagging deals can inhibit forecasting, too. Management could equally be to blame for not enforcing milestones or setting standards, as well as for not giving realistic sales projections or closing dates for current deals.
Sales forecasting is a challenge that’s ready for a data science solution. An example is IBM’s Budget, Authority, Need, and Time frame (BANT) process. BANT is IBM’s lead-scoring method. With this type of pipeline forecasting, each opportunity is fit into a stage of the sales process.
Then, a percentage generates a probability-adjusted revenue prediction. This lets IBM build forecasts using data from four inputs: the dollar amount, stage, probability, and close date. It’s an ideal approach for companies with long, multistage sales processes.
But this model doesn’t fit every business. For example, you may have concentrated revenue from a single account that gives a steady stream of deals. Tracking each individual deal doesn’t make sense. Instead, tracking from an account level may reveal seasonal or other trends in the volume of business that make for better forecasting.
On the other hand, you may have a large volume of smaller accounts in which tracking the progress of each deal is a wasted effort. Instead, you’d be better off just measuring the outcomes in aggregate and looking for overall trends.
Although it may be hard to forecast sales, data science can help. Capturing, reporting, and analyzing the right information lets sales leaders focus their efforts on the right sales prospects and find more missed opportunities.
Long-term Profitability
Profitability and the customer experience are intertwined. Rather than having an end-to-end experience that benefits customers, most businesses are set up to run as efficiently as possible from the supply and fulfillment side. Not only does this leave customers out of the equation, it may even make their lives more inconvenient. To create long-term revenue, companies must look at the customer’s experience from every single point of customer engagement.
A process for creating the most positive customer experience is referred to as customer journey mapping. This process lets you map the customer journey – from their first impressions to a purchase, the delivery, and all the way through to repeat sales.
While most companies are swimming in data, they don’t know how to translate it into a more positive customer experience. Data science can help.
Bonus: Improve the Customer Experience
We’re calling this point a bonus, because it’s very much tied to the previous points. If you start using data science in the right way to make the other changes, you almost can’t help but uncover things and make decisions that will ultimately improve the customer experience, too.
To make the most of this unified theory of marketing using data science, first you evaluate data from each touch point in your customer’s journey. Then, let the data show you how to improve customer experience in, for example:
- Call center conversations
- Social media inputs
- Customer questions or concerns that appear consistently
- Other areas that need improvement
By looking beyond the individual transaction to the complete journey, you can address the root causes and improve interactions upstream and downstream. Not only that, you can maximize your brand impact by aligning each touch point with your brand promise.
Of course, this will take new ways of gathering and analyzing your customer data. Sifting through the data with data science lets you uncover patterns that might take years to detect otherwise. This information will enhance both customer and employee satisfaction, thereby reducing customer churn and employee turnover. The result will be long-term increased revenues.
In very little time, data science can provide new knowledge that makes you better able to know what customers want from their experiences.
The Future Will Be Data Driven
With the growing use of digital marketing, it won’t be long before everything is connected digitally in some way. Data science gives you the tools to use the vast amounts of data you may already be sitting on.
But you need to ask the difficult leadership questions about marketing efficiency, return on investment, short-term revenue, and long-term profitability without getting bogged down. Sure, you can still do many things manually. But when all your competitors are using data science, will you be able to keep up?
Through determination to transform digitally, CEOs, CFOs, and other organizational leaders can use advanced data science tools and methods to make their collective vision a reality and lead their company into the future. Since there’s no doubt the future will be data driven, isn’t it better to get in the game sooner rather than later?