Step-by-Step Guide to Conducting a Successful AI Feasibility Study
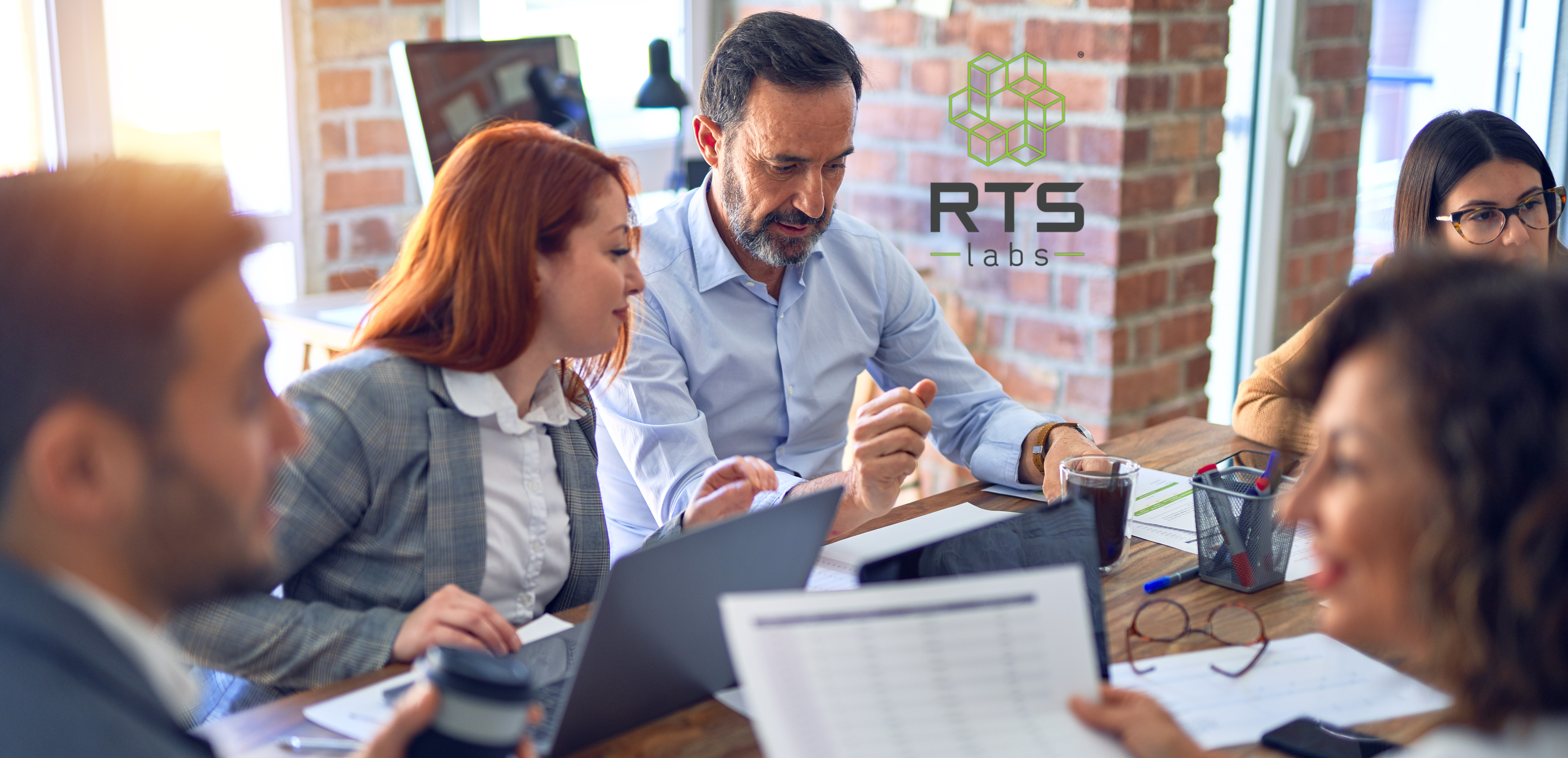
- Discover a quick guide to conducting a successful AI feasibility study for AI implementation
- Key steps include defining objectives, assessing data readiness, evaluating technical feasibility, analyzing organizational impact, and addressing ethical concerns
- Develop a prioritized roadmap and implementation plan based on feasibility study findings to ensure AI success
Artificial intelligence (AI) is revolutionizing the way businesses operate, but the path to successful implementation is not always clear. Many organizations find themselves at a crossroads, wondering if AI is right for their unique needs and challenges. This is where an AI feasibility study comes into play – a critical process that helps companies assess the viability, benefits, and risks of an AI project before committing valuable resources.
In this comprehensive guide, we’ll walk you through the essential steps of conducting an effective AI feasibility study. By following this systematic approach, you’ll be equipped to make informed decisions, align AI initiatives with business goals, and lay the foundation for a successful AI implementation.
Step 1: Define the Problem and Set Clear Objectives
The first and most crucial step in any AI feasibility study is to clearly define the problem you aim to solve and set specific, measurable objectives for the project.
Identifying the Problem
- Engage with key stakeholders across departments to understand their pain points and challenges
- Analyze existing processes, workflows, and data sources to pinpoint inefficiencies or areas for improvement
- Prioritize problems based on their impact on business goals, customer satisfaction, and operational efficiency
Setting SMART Objectives
- Define Specific, Measurable, Achievable, Relevant, and Time-bound (SMART) objectives for the AI project
- Align objectives with overall business strategy and KPIs
- Establish clear success metrics and performance indicators to track progress and measure ROI
Step 2: Assess Data Readiness and Quality
AI systems rely heavily on data to learn, adapt, and make accurate predictions or decisions. Therefore, evaluating the availability, quality, and relevance of your organization’s data is a critical component of the feasibility study.
Data Inventory and Assessment
- Conduct a comprehensive inventory of existing data assets, including structured and unstructured data sources
- Assess data quality in terms of accuracy, completeness, consistency, and timeliness
- Identify data gaps and determine if additional data collection or acquisition is necessary
Data Governance and Security
- Review current data governance policies and practices to ensure compliance with regulations and industry standards
- Evaluate data security measures and access controls to protect sensitive information
- Develop a data governance framework that aligns with the AI project’s requirements and objectives
Step 3: Evaluate Technical Feasibility and Infrastructure Requirements
Assessing the technical feasibility of an AI project involves understanding the computational resources, software tools, and infrastructure needed to support the development, training, and deployment of AI models.
AI Tools and Platforms
- Research and compare various AI tools, frameworks, and platforms based on their capabilities, scalability, and ease of use
- Consider factors such as community support, documentation, and integration with existing systems
- Evaluate the need for custom development versus off-the-shelf solutions
Infrastructure and Scalability
- Assess current IT infrastructure and identify any gaps or limitations in terms of storage, processing power, and network bandwidth
- Determine the scalability requirements for the AI system based on expected data volume, user traffic, and performance needs
- Consider cloud-based solutions or hybrid architectures for flexibility and cost-efficiency
Step 4: Analyze the Organizational Impact and Readiness
Implementing AI is not just a technical challenge; it also requires significant organizational change and alignment. Assessing your organization’s readiness and the potential impact of AI on people, processes, and culture is essential for a successful feasibility study.
Skills and Talent
- Evaluate the current skills and expertise of your workforce in relation to AI development, deployment, and maintenance
- Identify skill gaps and develop a plan for training, upskilling, or hiring specialized talent
- Foster a culture of continuous learning and innovation to adapt to the evolving AI landscape
Change Management
- Assess the potential impact of AI on existing roles, workflows, and decision-making processes
- Develop a change management strategy to communicate the benefits, address concerns, and ensure smooth adoption
- Engage employees in the AI journey through workshops, feedback sessions, and participatory design
Step 5: Evaluate the Ethical and Legal Implications
As AI systems become more sophisticated and autonomous, it is crucial to consider the ethical and legal implications of their use. A comprehensive feasibility study should address these concerns to ensure responsible and compliant AI deployment.
Fairness and Bias
- Assess the potential for bias in the data, algorithms, or decision-making processes
- Develop strategies to mitigate bias and ensure fairness, transparency, and accountability
- Regularly monitor and audit AI systems for any unintended consequences or discriminatory outcomes
Privacy and Security
- Ensure compliance with data protection regulations such as GDPR, CCPA, or industry-specific guidelines
- Implement robust security measures to safeguard sensitive data and protect against cyber threats
- Establish clear policies for data collection, storage, and usage in the context of AI applications
Step 6: Develop a Roadmap and Implementation Plan
Based on the findings of the feasibility study, the final step is to develop a comprehensive roadmap and implementation plan for the AI project.
Prioritization and Phasing
- Prioritize AI use cases based on their impact, feasibility, and alignment with business goals
- Break down the implementation into manageable phases or milestones
- Assign resources, budgets, and timelines for each phase of the project
Monitoring and Iteration
- Establish a framework for continuous monitoring and evaluation of the AI system’s performance and outcomes
- Plan for regular iterations and updates based on user feedback, changing requirements, or technological advancements
- Foster a culture of experimentation and learning to continuously improve the AI solution
Embarking on Your AI Journey with Confidence
Conducting a thorough AI feasibility study is a critical first step in ensuring the success of your AI initiatives. By following the step-by-step approach outlined in this guide, you’ll be well-equipped to assess the viability, benefits, and risks of AI projects in your organization.
Remember, an AI feasibility study is not a one-time exercise but an ongoing process that requires continuous evaluation and adaptation. As the AI landscape evolves and new opportunities emerge, regularly revisiting and updating your feasibility study will help you stay ahead of the curve and make informed decisions.
By investing time and resources in a comprehensive AI feasibility study, you’ll lay the foundation for a successful AI implementation that drives business value, improves operational efficiency, and unlocks new opportunities for growth and innovation.
So, embrace the power of AI with confidence, knowing that you’ve taken the necessary steps to ensure a successful and impactful AI journey for your organization.
FAQs: Your AI Feasibility Study Questions, Answered
Q: Why is an AI feasibility study important?
A: An AI feasibility study is crucial because it helps your organization determine if an AI project is a good fit. It assesses whether the project is technically possible, aligns with your goals, and will deliver a positive return on investment. It also helps you identify potential risks and challenges upfront.
Q: Who should be involved in an AI feasibility study?
A: A successful feasibility study requires a cross-functional team. Include stakeholders from various departments (IT, business units, legal, etc.), data scientists, and potentially external AI consultants. Each brings unique perspectives and expertise.
Q: How long does an AI feasibility study typically take?
A: The duration of a feasibility study varies depending on the complexity of the project, the size of your organization, and the resources available. It can range from weeks to months.
Q: What are the key risks to consider in an AI feasibility study?
A: Key risks include:
- Data issues: Insufficient data, poor data quality, or data bias.
- Technical challenges: Lack of appropriate infrastructure, difficulty integrating AI with existing systems, or choosing the wrong AI tools.
- Organizational resistance: Employees may be hesitant to adopt new technology or concerned about job displacement.
- Ethical concerns: AI systems can perpetuate bias or raise privacy concerns.
Q: What happens after an AI feasibility study?
A: If the study concludes that AI is feasible, the next steps are to develop a detailed implementation plan. This includes selecting the right AI technology, creating a timeline, allocating resources, and managing the change within your organization.
Q: Can I conduct an AI feasibility study myself, or should I hire a consultant?
A: While you can conduct a basic study internally, complex projects often benefit from the expertise of AI consultants. They can provide unbiased assessments, technical guidance, and best practices from other implementations.
Q: What are some common mistakes to avoid in an AI feasibility study?
A:
- Overlooking data: Data is the foundation of AI. Don’t underestimate the importance of having sufficient, high-quality data.
- Ignoring organizational impact: AI can disrupt workflows and roles. Involve employees early to address concerns and build support.
Focusing only on technology: Remember that AI is not just about the tools; it’s about solving real business problems.