Revolutionizing Green Logistics: The Power of AI in Route Optimization
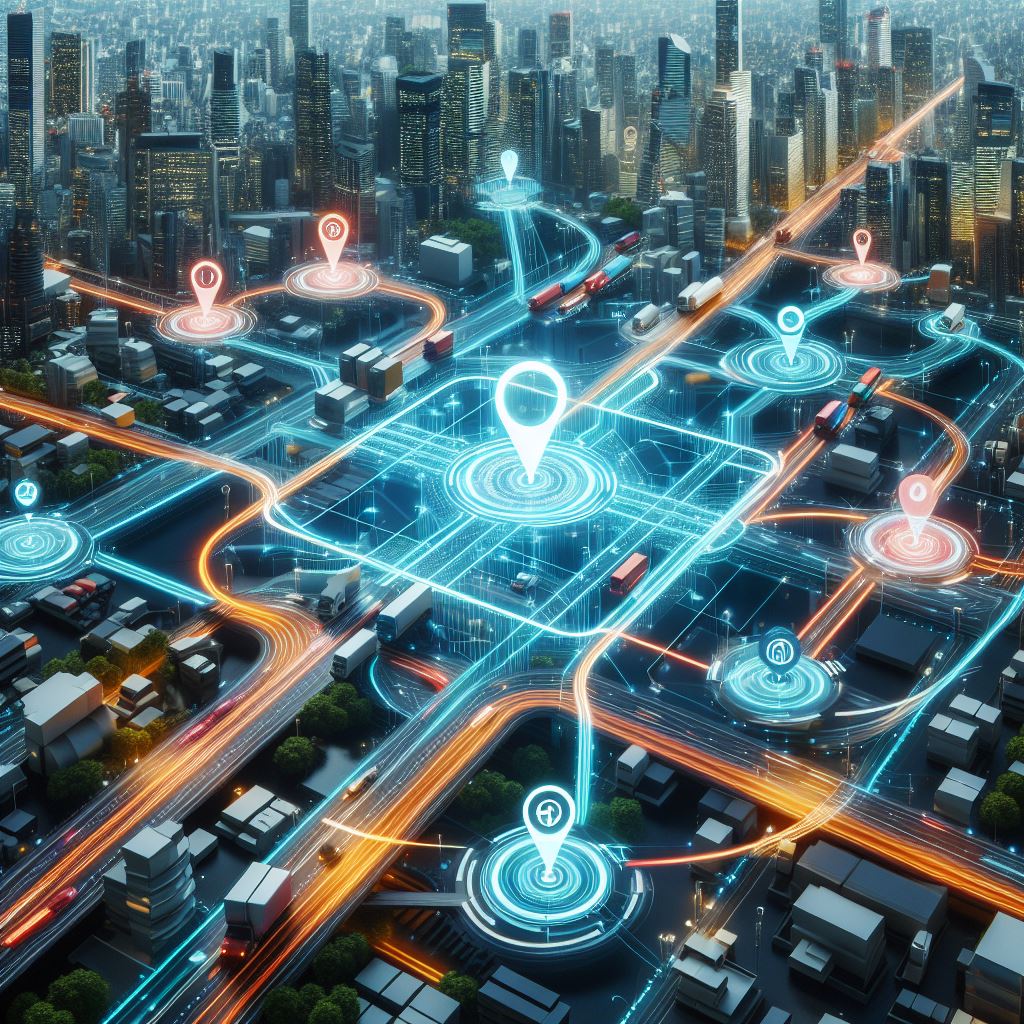
How AI Can Help Logistics Companies Reduce Their Environmental Impact
In modern transportation, managing and maintaining efficiency is critical to ensure consumer satisfaction while simultaneously reducing environmental damage. AI comes up with novel solutions to these problems. AI can help transportation businesses reduce their environmental impact by increasing efficiency, predictive analytics, and making smarter decisions.
Because transportation is so crucial in global trade, there is a lot of pressure on the industry to go green. Transport is under pressure to improve due to its significant contribution to global carbon emissions. AI is a great tool for sustainability because it can analyze large amounts of data and forecast future outcomes.
Route Optimization: A Closer Look
Route optimization stands as a major application of Artificial Intelligence (AI) in logistics, aimed at enhancing environmental sustainability while maintaining operational efficiency. By leveraging advanced algorithms, logistics companies can minimize fuel consumption and emissions, contributing significantly to their green initiatives. This section delves deeper into the mechanics and benefits of route optimization.
How AI Enables Route Optimization
AI is making logistics route planning more dynamic, efficient, and adaptable to real-time changes. Logistics operations can design and execute delivery routes with unparalleled accuracy and efficiency using AI. AI makes this transformation possible:
Data Analysis
AI algorithms ingest a vast array of data, including historical traffic patterns, real-time road conditions, weather forecasts, and vehicle performance metrics. By analyzing this data, AI can identify patterns and predict potential bottlenecks or delays.
Efficient Pathfinding
Utilizing machine learning techniques, AI algorithms can calculate the most efficient routes for delivery vehicles. These routes are not solely based on distance but also consider factors like traffic congestion, road closures, and delivery time windows.
Dynamic Adjustments
AI systems can continuously monitor route conditions and make real-time adjustments. For instance, if an unexpected traffic jam occurs, the system can reroute the vehicle to avoid delays, ensuring timely deliveries with minimal fuel wastage.
Vehicle Load Optimization
Beyond route planning, AI can also optimize vehicle loading. By strategically packing items based on their delivery sequence and weight distribution, fuel efficiency is further enhanced.
Benefits of Route Optimization
AI in route optimization improves operational efficiency, environmental sustainability, and cost savings. AI lets logistics organizations improve delivery routes in ways never before possible using powerful algorithms and machine learning. AI route optimization offers certain advantages:
Reduced Fuel Consumption
By identifying the shortest and least congested routes, AI-enabled route optimization significantly lowers fuel usage. This not only cuts operational costs but also reduces greenhouse gas emissions, aligning with environmental sustainability goals.
Decreased Carbon Footprint
Reduced fuel consumption directly translates to lower carbon emissions. Logistics companies can thus contribute to mitigating climate change and adhere to regulatory requirements concerning emissions.
Enhanced Delivery Efficiency
Optimized routes lead to faster deliveries, improving customer satisfaction and enabling companies to handle a higher volume of orders with the same fleet size.
Cost Savings
Fuel is a major expense in logistics. By minimizing fuel usage, companies can achieve substantial cost savings, which can be redirected toward other sustainability initiatives or business development.
Scalability
AI-based route optimization can easily scale to accommodate growing fleets and expanding delivery networks, making it a sustainable solution for long-term growth.
Case Study: UPS
UPS, a global logistics giant, has been a frontrunner in adopting AI for route optimization. Their proprietary system, ORION (On-Road Integrated Optimization and Navigation), analyzes over a billion data points daily to optimize delivery routes. Since its implementation, UPS has reported saving over 10 million gallons of fuel annually and reducing its carbon emissions by over 100,000 metric tons each year. These savings underscore the tangible environmental and economic benefits of AI-driven route optimization in the logistics sector.
Route optimization is a testament to the power of AI in revolutionizing logistics operations while prioritizing environmental sustainability. By intelligently planning routes, logistics companies can significantly reduce their ecological footprint, save costs, and enhance service efficiency. As AI technology continues to evolve, its role in fostering greener logistics practices is expected to grow, paving the way for a more sustainable future in the industry.
Fleet Management: An In-Depth Analysis
Fleet management is a critical component of logistics operations, and the integration of Artificial Intelligence (AI) has revolutionized this domain. AI-driven systems enhance the efficiency and sustainability of fleet operations by providing real-time monitoring, predictive maintenance, and strategic vehicle composition recommendations. This section explores the intricacies of AI in fleet management and its environmental implications.
Real-Time Vehicle Health Monitoring
Modern vehicles are equipped with an array of sensors that collect data on engine performance, fuel consumption, tire pressure, and more. AI systems analyze this data in real time to assess vehicle health and performance. These algorithms can identify deviations from normal performance patterns, signaling potential issues. For example, a sudden increase in fuel consumption could indicate a problem with the engine or exhaust system. By detecting anomalies early, AI-driven systems can alert fleet managers to potential maintenance needs before they escalate into serious problems.
This proactive approach minimizes downtime and prevents costly repairs. A study found that the use of AI in commercial vehicles resulted in 22% fewer accidents and 56% fewer unsafe driving incidents across 5,000 fleets.
Predictive Maintenance
AI algorithms can predict when a vehicle is likely to require maintenance based on historical data and current performance metrics. This enables fleet managers to schedule maintenance in advance, avoiding unexpected breakdowns. By accurately predicting maintenance needs, AI systems can extend the intervals between repairs, ensuring that vehicles are not taken out of service unnecessarily. This optimizes the utilization of each vehicle in the fleet and reduces the likelihood of major repairs, leading to significant cost savings over time. A McKinsey study states that predictive maintenance can reduce costs by 10-40%, downtime by 50%, and capital investment by 3-5%.
Fleet Composition Optimization
AI can analyze the specific needs of a logistics operation, such as delivery routes, cargo types, and environmental goals, to recommend the most suitable vehicles for the fleet. This ensures that each vehicle is well-suited to its intended tasks. AI systems can identify opportunities to integrate electric or hybrid vehicles into the fleet, considering factors such as charging infrastructure availability, route distances, and payload capacities. By calculating the potential reduction in emissions and fuel consumption resulting from the adoption of electric or hybrid vehicles, AI provides a clear picture of the environmental benefits.
Inventory Management: A Comprehensive Overview
Inventory management is a fundamental aspect of logistics operations, and its efficiency has a direct impact on environmental sustainability. The advent of Artificial Intelligence (AI) in this domain has enabled logistics companies to achieve unprecedented levels of accuracy and efficiency, significantly reducing waste and emissions. This section delves into the role of AI in enhancing inventory management practices.
Demand Prediction and Stock Optimization
One of the primary contributions of AI to inventory management is its ability to predict demand with remarkable precision. By analyzing historical sales data, market trends, and external factors such as seasonal variations and economic indicators, AI algorithms can forecast future demand for products. This predictive capability allows companies to maintain optimal stock levels, avoiding the pitfalls of overstocking and understocking.
Overstocking leads to excess inventory that may eventually become obsolete, resulting in waste and financial losses. Understocking, on the other hand, can lead to stockouts, disappointing customers and potentially driving them to competitors. By striking the right balance, AI-driven inventory management minimizes these risks so that the products are available when needed without excessive surplus.
Reducing Emergency Shipments
Emergency shipments are often required to replenish stock quickly when unexpected shortages occur. These shipments typically rely on expedited transportation modes, such as air freight, which have a significantly higher carbon footprint compared to slower, more efficient methods like sea or rail transport. By accurately predicting demand and maintaining optimal stock levels, AI reduces the reliance on emergency shipments, thereby decreasing the environmental impact associated with logistics operations.
Energy Efficiency in Warehouses
AI plays a crucial role in optimizing stock levels, which in turn influences the energy consumption of warehouses. Excess inventory requires additional storage space, leading to higher energy usage for lighting, heating, cooling, and maintaining the facility. AI helps reduce the energy footprint of warehouses by keeping inventory levels in check.
Furthermore, AI can assist in the strategic layout of warehouses, ensuring that frequently accessed items are placed in easily accessible locations. This reduces the time and energy required for picking and packing processes, contributing to overall energy efficiency.
AI-driven inventory management is a key enabler of sustainability in logistics operations. By accurately predicting demand, optimizing stock levels, reducing the need for emergency shipments, and enhancing energy efficiency in warehouses, AI significantly reduces waste and emissions. As logistics companies continue to embrace AI in inventory management, the environmental benefits are expected to grow, contributing to a more sustainable and efficient supply chain.
Predictive Analytics for Sustainable Packaging: An In-Depth Exploration
Sustainable packaging has emerged as a critical focus area in the logistics and consumer goods industries, driven by increasing environmental awareness and consumer demand for eco-friendly practices. Artificial Intelligence (AI) plays a pivotal role in advancing sustainable packaging initiatives through predictive analytics. This section examines how AI contributes to the development and implementation of sustainable packaging solutions.
Understanding Consumer Preferences
AI algorithms can analyze vast amounts of customer feedback, social media conversations, and market research data to gauge consumer preferences for sustainable packaging. By identifying trends and patterns in consumer behavior, companies can tailor their packaging strategies to align with eco-conscious values. This consumer-centric approach ensures that sustainability efforts resonate with the target audience, enhancing brand reputation and customer loyalty.
Predicting Product Suitability for Eco-Friendly Packaging
Not all products are equally suited for sustainable packaging due to factors such as shelf life, fragility, and regulatory requirements. AI can assess product characteristics and market dynamics to predict which items are most likely to benefit from eco-friendly packaging. For example, products with a shorter shelf life might be ideal candidates for biodegradable packaging, while durable goods could be paired with reusable or recyclable options.
Material Selection and Innovation
AI-driven predictive analytics can aid in the selection of sustainable materials for packaging. By analyzing data on material properties, environmental impact, cost, and supply chain logistics, AI can recommend materials that balance sustainability with practicality and economic viability. Additionally, AI can facilitate innovation in packaging design, identifying opportunities to reduce material usage, enhance recyclability, and improve overall environmental performance.
Optimizing Packaging for Efficiency and Waste Reduction
AI can optimize packaging design to minimize waste and reduce the environmental footprint. By analyzing product dimensions, shipping requirements, and packaging materials, AI algorithms can suggest design modifications that decrease excess packaging, improve space utilization in transportation, and enhance the recyclability of the packaging.
Life Cycle Assessment and Impact Analysis
AI can perform life cycle assessments of packaging options, evaluating their environmental impact from production to disposal. This comprehensive analysis helps companies understand the true sustainability of different packaging choices, enabling informed decision-making that prioritizes long-term environmental benefits.
Enhancing Supply Chain Transparency
Supply chain transparency is becoming essential to sustainable logistics and ethical business. As environmental concerns rise, firms are seeking deeper supply chain analytics to detect and solve environmental hazards. AI can improve openness, decision-making, and accountability. Artificial intelligence’s role in supply chain transparency is as follows:
Real-Time Tracking and Visibility
AI-powered systems provide comprehensive tracking of products and materials, integrating data from GPS trackers, sensors, and supply chain management software. This capability allows for monitoring the environmental impact of logistics and identifying areas for sustainable practice improvements.
Carbon Footprint Calculation
AI algorithms calculate the carbon emissions at each step of the supply chain, analyzing data on energy consumption, transportation modes, and production processes. This aids in pinpointing environmental impact hotspots.
Supplier Assessment and Selection
AI tools evaluate suppliers based on their sustainability practices, analyzing energy usage, waste management, and regulatory compliance to recommend partners that align with sustainability goals.
Predictive Analytics for Risk Management
AI’s predictive analytics forecast potential supply chain disruptions, such as natural disasters or regulatory changes, enabling proactive risk management.
Enhanced Reporting and Accountability
AI-generated data supports accurate and detailed reporting on supply chain sustainability, promoting transparency with stakeholders, regulatory bodies, and customers.
Energy Efficiency in Warehouses: A Comprehensive Exploration
Warehouses store and distribute commodities, making them vital to the logistics chain. They also use a lot of energy for lighting, heating, ventilation, and cooling, which increases their operating expenses and environmental effects. AI in warehouse operations can improve energy efficiency, slash expenses, and minimize logistics’ carbon impact.
Key ways AI improves warehouse energy efficiency include:
Optimizing Warehouse Layouts
AI algorithms can design layouts that minimize energy consumption by analyzing the flow of goods, worker movement patterns, and equipment usage.
Smart Lighting Systems
AI-powered systems adjust lighting levels based on natural light availability, occupancy, and specific tasks, significantly reducing electricity usage.
HVAC Optimization
AI optimizes HVAC systems by dynamically adjusting temperature and ventilation settings based on external weather conditions, internal conditions, and occupancy patterns.
Renewable Energy Management
AI ensures that renewable energy systems, like solar panels, operate at maximum efficiency, reducing reliance on fossil fuels.
Predictive Maintenance
By monitoring equipment and predicting maintenance needs, AI prevents unexpected breakdowns and ensures systems operate at peak efficiency.
Conclusion
As logistics companies strive to balance efficiency, customer satisfaction, and environmental sustainability, Artificial Intelligence (AI) emerges as a powerful tool to address these challenges. AI’s capabilities in route optimization, predictive maintenance, and inventory management significantly reduce the environmental impact of logistics operations. By optimizing routes, AI minimizes fuel consumption and emissions, while predictive maintenance ensures vehicles operate at peak efficiency, further reducing their carbon footprint. Additionally, AI-driven inventory management reduces waste and energy usage in warehouses. As AI technology continues to evolve, its role in promoting sustainable logistics practices is expected to grow, paving the way for a greener and more efficient future in the industry.Â