Functional Examples of Predictive Analytics in Supply Chain Management
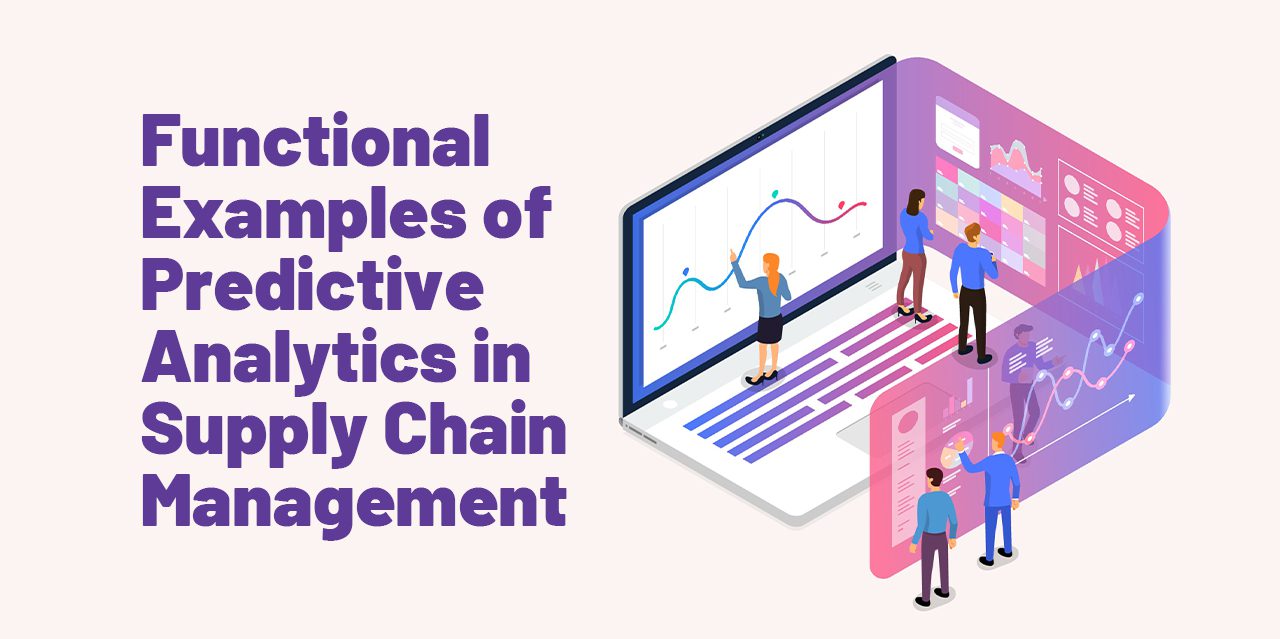
Supply chain management is a complex process that benefits from being carefully planned and executed. It increases the odds of success and keeps everything manageable for everyone involved.
One smart tool to aid in the process is predictive analytics. Analyzing data can help businesses make better decisions based on supply chain management.
This blog post will provide examples of how predictive analytics is used in supply chain management and discuss the advantages of predictive analytics in the first place.
The Importance of Supply Chain Predictive Analytics
Predictive analytics is essential for supply chain management. Predicting future demand allows companies to ensure they have the right amount of inventory on hand, preventing stock-outs and making sure their customers always have what they need.
Even though predictive analytics is not a crystal ball, they provide businesses with a significant competitive edge. A company that embraces predictive analytics will thrive in the digital age.
Functional Examples of Predictive Analytics
Businesses use predictive analytics to make better decisions about inventory, production, and delivery through supply chain management. The use of predictive models allows companies to identify patterns and trends that would otherwise be invisible.
Businesses can utilize this information to optimize their supply chains and provide better customer service. For instance, a retailer might use predictive analytics to predict demand for a certain product. If a retailer anticipates that demand will increase, it can order more of the product from its suppliers.
Consider what you would do if you ran a store that sold women’s clothing. A predictive analytics tool might help predict demand for skirts during specific times of the year. In the spring, you can order more skirts from your suppliers if you anticipate an increase in demand.
Here is another way to look at it: imagine you are a transportation company that delivers goods across the country. Predictive analytics can be used to determine which routes are most likely to experience traffic congestion. Using this information, you can plan your deliveries accordingly.
Another example is a transportation company that uses predictive analytics to determine what routes are most likely to experience traffic congestion. Knowing this information can help the company better plan their deliveries.
If the company knows that a certain route is likely to be congested on a particular day of the week, it might send a different truck down that route. By doing so, they can avoid having delays and keep their supply chain running smoothly.
Additionally, predictive analytics is useful for identifying potential supply chain problems. If a company is experiencing a shortage of a particular component, predictive analytics can help identify the cause.
Oftentimes, it’s simply a spike in demand, shrinkage, or a break in the chain leading to communications. Predictive analytics can help narrow down the causes and shed light on what would otherwise just be speculation.
Supply Chain Predictive Analytics Tips
Predictive Analytics Tip #1: Make Sure You Have Accurate Data
The first step in any predictive analysis is to gather accurate data. Without good data, your predictions will not be very accurate.
This means ensuring there is no conflicting information in your data. If you notice there are some inconsistencies throughout, it’s safe to say something along the supply chain isn’t being reflected properly.
Still, it’s a relatively easy fix. It just involves taking a deeper look at the values that aren’t lining up. You might find a common cause is a simple miscommunication, but it’s better to resolve it now than later.
Predictive Analytics Tip #2: Use Historical Data to Train Your Models
The best way to create accurate predictive models is to use historical data to train them. This means using historical values, numbers you know you can count on. Accurate numbers that showcase all sides of the supply chain.
Also, be sure to include as much relevant data as possible so that your models will be able to accurately predict the future. Don’t just focus on the obvious, add some context around your information.
Predictive Analytics Tip #3: Use Multiple Models
Predictive analytics is an inexact science, and no single model will be accurate in all cases. Therefore, it’s important to use multiple models to get a more holistic view of the data.
Try using models that focus on specific aspects of the supply chain, as well as some that have a more broad view of all of it. Just like a good argument, it’s important to look at all sides of any given situation.
Predictive Analytics Tip #4: Test Your Models
Once you have created predictive models, it’s important to test them to see how accurate they are. You can do this by comparing the predictions of the models against actual outcomes.
Odds are, they won’t always line up—and that’s fine. After all, predictive models are meant to be educated guesswork. The goal here is to make sure they’re as accurate as possible so you can realistically predict outcomes later.
Predictive Analytics Tip #5: Use Feedback to Improve Your Models
Once you’ve tested your predictive models, you can use the feedback to improve them. This will help make them even more accurate in the future.
Remember, this may mean a few, or several tweaks. It all depends on how accurate your analytics were in the first place. Still, carve out the time to handle this upfront, and you’ll get tons of value later.
Predictive Analytics Tip #6: Keep Track of Your Predictions
It’s important to keep track of the predictions that your predictive models make, so you can track their accuracy over time.
Don’t be discouraged if they’re very inaccurate to start. A common arc you might see is that they start off fairly inaccurate, but slowly transform into super helpful, highly accurate models. It can happen in the span of a few months, depending on how accurate they were to start with.
Predictive Analytics Tip #7: Use Predictive Analytics in Conjunction With Other Supply Chain Tools
Predictive analytics can be a powerful tool, but it should be used in conjunction with other supply chain tools to get the best results.
Supply and demand analysis, inventory management, supplier management, and shipping and delivery are all important factors, and there are lots of tools available to measure them. With the competitive nature of business today, several analytics tools are now adding these features into their platform for ease of use.
Predictive Analytics Tip #8: Don’t Rely On Predictions Alone
Although predictive analytics can be a valuable tool, it should not be used to make decisions in isolation. Always use your judgment, along with the predictions of your models, to make the best decision possible.
If you find yourself relying heavily on predictive analytics, it might be a good idea to bring in more of your team into the process. Different viewpoints and opinions can shed light on areas you might not have otherwise uncovered yourself.
Predictive Analytics Are Essential for Supply Chain Management
To summarize, predictive analytics is a powerful tool that can be utilized in many ways to increase supply chain performance. Predictive analytics allows supply chain managers to make better decisions, reduce costs, and prevent disruptions.
Looking for the right software development partner? RTS Labs has helped hundreds of businesses of all sizes successfully develop the right outsourced custom software for many business needs. Get in touch with us today to learn more about how we can help.