Navigating Risk: AI’s Transformative Role in Finance
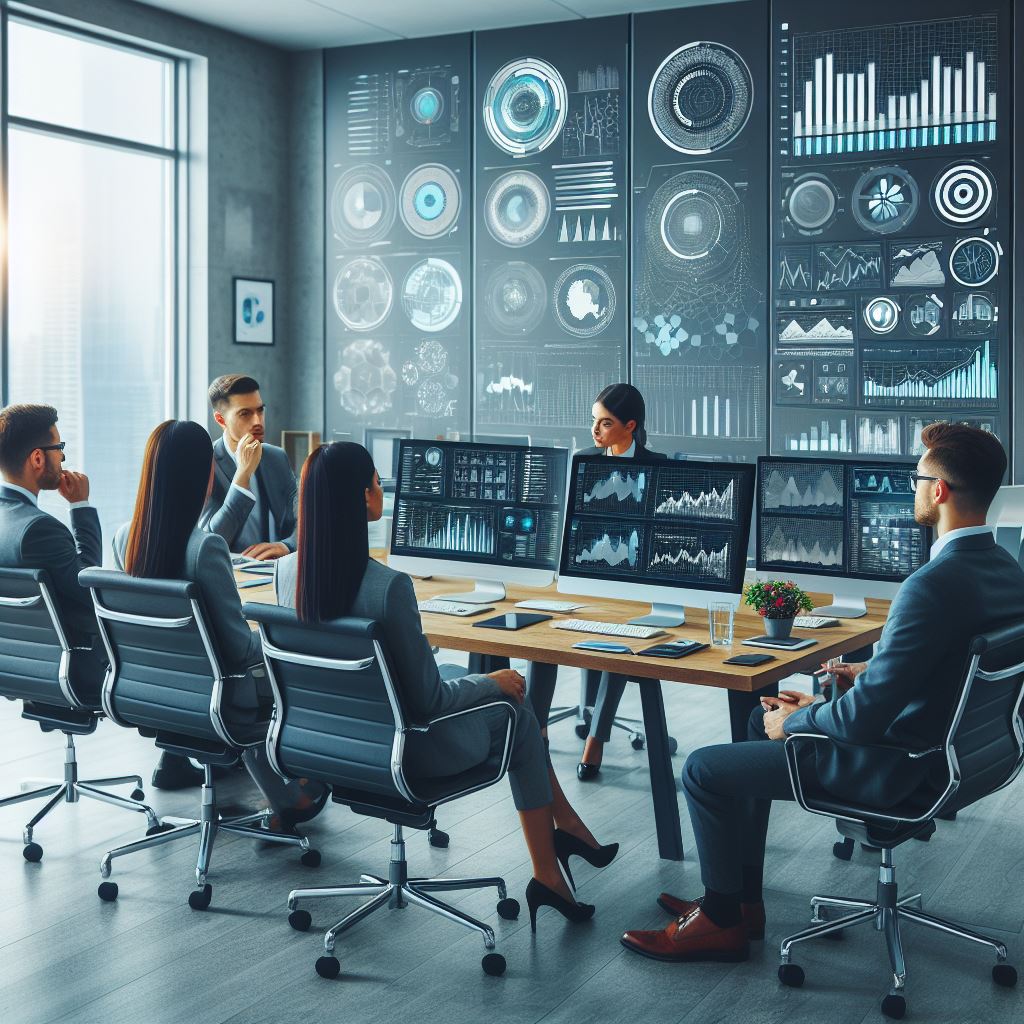
The Benefits of Using AI in Risk Management
In today’s complex business environment where challenges like evolving regulations, adoption of hybrid work models, and rapid technological changes are the norm, organizations need to fortify themselves against the ever-changing risk landscape. Establishing strong risk management programs becomes crucial to shield businesses from potential cyber threats.
According to a recent survey, 35% of risk executives see compliance and regulatory risks, along with other operational challenges, as the most significant threats to their company’s growth. Another 35% express concern about cyber or information risks. Additionally, a substantial 61% of risk executives highlight data protection and privacy regulations as their companies’ top priorities in 2022.
As businesses tackle the complexities of our ever-changing landscape, AI becomes a key player, amplifying our ability to grasp, foresee, and address dynamic risks. This case study aims to provide real-world insights into how AI acts as a game-changer in risk management.
AI in Risk Identification and Mitigation
Traditional risk identification methods face several challenges in the current business landscape. Firstly, these methods often rely on historical data and predetermined rules, making it challenging to adapt to the dynamic and evolving nature of risks. Additionally, traditional approaches struggle to analyze the vast amount of data generated in real-time, hindering their ability to detect emerging threats promptly. The lack of predictive capabilities and the manual nature of these methods further limit their effectiveness in addressing modern challenges, including rapidly changing regulations, cyber threats, and operational risks.
Artificial Intelligence (AI) brings a transformative paradigm to risk detection by leveraging predictive analytics and machine learning models. Unlike traditional methods, AI operates in real-time, enabling organizations to analyze large and dynamic datasets on the fly.
Predictive analytics allows AI systems to identify patterns, trends, and anomalies, contributing to a proactive risk detection approach. Machine learning models, through continuous learning and adaptation, enhance the system’s ability to recognize and understand emerging risks, even those previously unseen.
Case Study: RTS Labs – Revolutionizing Risk Mitigation with AI
Problem
RAZE Banking, a prominent financial institution, faced escalating challenges in risk mitigation. Traditional methods struggled to keep pace with the evolving landscape of cyber threats, compliance issues, and operational risks. The bank experienced a surge in fraudulent activities, leading to financial losses and compromising its reputation.
Solution
RTS Labs, renowned for its expertise in AI solutions, collaborated with RAZE Banking to implement a cutting-edge risk mitigation strategy. The team at RTS Labs designed a sophisticated AI system that incorporated predictive analytics and machine learning models. This system seamlessly integrated with the bank’s existing infrastructure, enabling real-time analysis of transactions, customer behavior, and regulatory compliance.
Implementation
Predictive Analytics
The AI system utilized predictive analytics to assess historical data and identify potential patterns indicative of fraudulent activities. This proactive approach allowed the bank to anticipate risks before they could manifest.
 Machine Learning Models
The machine learning models continuously learn and adapt to new data, enhancing their ability to recognize evolving threats. These models could discern subtle anomalies in transaction patterns, flagging potential risks with precision.
Results
Fraud Prevention
Within the first three months of implementation, RAZE Banking witnessed a remarkable 45% reduction in fraudulent transactions.
Regulatory Compliance
The AI system ensured adherence to data protection and privacy regulations, addressing a critical concern for the bank. This led to a 20% improvement in regulatory compliance efficiency.
 Operational Efficiency
With real-time risk analysis, the bank experienced a 30% increase in operational efficiency, streamlining processes and reducing manual intervention.
 Client Testimonial
“RTS Labs has been our strategic partner in fortifying our risk management efforts. The AI solution they implemented not only prevented financial losses but also brought a new level of confidence in our ability to navigate complex risks. It’s not just about technology; it’s about securing our future.” – Mr. John Anderson, CEO, ABC Banking.
AI in Regulatory Compliance
The finance sector experiences a myriad of regulatory challenges, navigating a landscape characterized by evolving standards, intricate compliance requirements, and the need for real-time adherence. Traditional methods often fall short of efficiently managing the complexity and volume of regulatory changes, exposing financial institutions to the risk of non-compliance and associated penalties.
Role of AI in Simplifying Compliance Processes
Artificial Intelligence (AI) emerges as a transformative force in simplifying compliance processes within the finance industry. AI facilitates enhanced data management, automating the sorting, categorization, and analysis of vast datasets. This not only reduces the manual workload but also ensures accuracy in interpreting regulatory requirements. Real-time monitoring, a critical aspect of compliance, is significantly bolstered by AI, allowing financial institutions to promptly identify and address potential compliance issues as they arise.
Examples of Financial Institutions Using AI for Compliance
Case Example 1: SmartBank Solutions
SmartBank Solutions, a forward-thinking financial institution, integrated AI into its compliance processes. The AI system continuously scans regulatory updates, assesses their impact on existing policies, and automatically implements necessary adjustments. This proactive approach ensures that SmartBank remains ahead of compliance requirements, mitigating the risk of non-compliance.
Case Example 2: SecureInvest Insights
SecureInvest Insights, a leading investment firm, leverages AI for real-time monitoring of transactions. The AI system swiftly identifies irregularities, ensuring adherence to anti-money laundering (AML) and Know Your Customer (KYC) regulations. This has not only streamlined compliance but also fortified SecureInvest’s reputation for maintaining a secure and compliant financial environment.
Impact on Adherence to Regulations and Ease of Compliance
The integration of AI into compliance processes has a profound impact on financial institutions. It enhances the accuracy and efficiency of adherence to regulations, reducing the likelihood of errors and ensuring timely adjustments to policy changes. The real-time capabilities of AI contribute to a proactive stance in compliance, offering an environment where financial institutions can navigate regulatory challenges with greater ease and confidence. The result is not only enhanced compliance but also a more resilient and agile response to the ever-evolving regulatory landscape.
AI in Improving Operational Efficiency
Navigating the intricate landscape of financial operations brings forth challenges such as manual workflows, resource allocation complexities, and the demand for swift adaptability. Financial institutions grapple with the need for streamlined processes that can efficiently handle high transaction volumes and dynamic market shifts.
Leverage of AI for Operational Streamlining
In the quest for operational excellence, financial institutions turn to Artificial Intelligence (AI) as a transformative ally. AI’s automation capabilities are harnessed to handle repetitive tasks, freeing up human resources and mitigating the risk of errors. Through process optimization, AI analyzes existing workflows, identifies bottlenecks, and proposes enhancements, contributing to an overall more efficient operational landscape.
Showcasing Increased Operational Efficiency through Case Studies
Case Example 1: Pinnacle Finance Dynamics
Pinnacle Finance Dynamics collaborated with RTS Labs to revamp its operational structure. The integration of AI-driven automation targeted tasks like data entry and transaction processing. The outcome was a remarkable 40% reduction in processing times, enabling Pinnacle Finance Dynamics to redirect resources toward strategic initiatives.
Case Example 2: Velocity Investments Solutions
Velocity Investments Solutions embraced AI for holistic process optimization. The AI system scrutinized the investment decision-making process, uncovering inefficiencies and suggesting improvements. This not only accelerated investment decisions by 30% but also elevated the accuracy of portfolio management, leading to an overall enhancement in operational efficiency.
Tangible Gains in Numbers and Quality
The adoption of AI to enhance operational efficiency yields both quantifiable and qualitative advantages. In quantitative terms, financial institutions witness substantial reductions in processing times, cost savings via automation, and optimized resource management. Qualitatively, there’s a noticeable improvement in process accuracy, a decrease in errors, and heightened adaptability to market dynamics.
AI in Driving Cost Reduction
Financial institutions operate in a landscape where managing costs is a perpetual challenge. Concerns encompass operational expenses, resource allocation, and the imperative to optimize financial processes to ensure sustainability and competitiveness.
Strategies Employed by AI for Cost Reduction
The strategic deployment of Artificial Intelligence (AI) emerges as a potent tool for mitigating cost concerns. AI contributes to cost reduction through error reduction, automating processes, and enhancing overall operational efficiency. By automating routine tasks and minimizing the risk of human errors, AI becomes a catalyst for substantial cost savings.
Real-Life Examples of Achieved Cost SavingsÂ
Case Example 1: PrecisionBank Solutions
PrecisionBank Solutions, in collaboration with RTS Labs, implemented AI-driven process automation. This initiative targeted manual tasks prone to errors, resulting in a 30% reduction in error-related costs. The streamlined processes not only increased accuracy but also led to notable cost savings.
Case Example 2: CapitalOptimize Strategies
CapitalOptimize Strategies integrated AI for cost-efficient resource allocation. Through predictive analytics, AI assessed resource needs, optimizing staffing levels based on demand fluctuations. The result was a 25% reduction in labor costs while maintaining operational excellence.
Long-Term Financial Impact of Integrating AI
The integration of AI into cost-reduction strategies yields a sustainable and long-term financial impact for financial institutions. Beyond immediate cost savings, AI contributes to increased operational efficiency, enabling institutions to allocate resources strategically. The long-term financial impact encompasses enhanced competitiveness, adaptability to market changes, and the ability to invest in innovative initiatives that drive further growth. As financial institutions navigate the dynamic economic landscape, AI proves to be not just a cost-saving tool but a strategic enabler of sustained financial health and resilience.
AI in Enhancing Business Decision-Making
Effective decision-making forms the bedrock of success in the financial realm. In a dynamic landscape marked by market shifts and regulatory changes, sound decisions are crucial for financial institutions to thrive, innovate, and stay resilient.
AI’s Role in Informed Decision-Making
Artificial Intelligence (AI) emerges as a game-changer in fostering informed decision-making. By harnessing the power of data analysis and predictive forecasting, AI provides financial institutions with insights that go beyond human capacity. The ability to process vast datasets swiftly allows for more accurate risk assessments, market trend analysis, and proactive decision-making.
Instances where AI-Led Decisions Benefited Institutions
Case Example 1: InsightfulInvest Dynamics
InsightfulInvest Dynamics implemented AI for data-driven decision-making. The AI algorithms analyzed market trends, assessed investment risks, and provided real-time insights. This resulted in a 20% increase in successful investments and minimized losses due to proactive decision-making.
 Case Example 2: RiskGuard Strategies
RiskGuard Strategies utilized AI for risk management decisions. The AI system processed a multitude of data points, enabling RiskGuard to identify potential risks early on. This proactive approach led to a 15% reduction in financial losses attributed to unforeseen market changes.
Overall Improvement in Business Outcomes
The incorporation of AI in decision-making processes contributes to a comprehensive improvement in business outcomes. Financial institutions witness enhanced accuracy in risk assessments, more effective allocation of resources, and the ability to seize opportunities swiftly. The ripple effect is an institution that not only navigates uncertainties adeptly but also positions itself for sustained growth and competitiveness in the ever-evolving financial landscape.
Conclusion
In the intricate world of finance, where challenges abound, Artificial Intelligence (AI) emerges as a trustworthy ally, paving the way for a more secure and resilient future. Our journey through AI’s role in risk management has shown that it’s more than just technology – it’s a transformative force shaping the very essence of financial institutions.
AI’s knack for identifying and mitigating risks goes beyond the norm, using predictive analytics and machine learning models as vigilant guardians against evolving threats. It’s not just about adapting; it’s about anticipating and fortifying financial landscapes with newfound precision.